Commonsense reasoning in ai involves teaching agents about the world through precise and accurate information. We will explore the importance of commonsense reasoning in ai and how it enhances the capabilities of ai agents to understand and navigate the world around them.
By incorporating commonsense reasoning, ai systems can make more informed decisions, interact with humans more effectively, and adapt to dynamic environments. This article will delve into the advancements and challenges in teaching ai agents about the world, as well as the potential future implications of commonsense reasoning in ai.
So let’s dive in and explore the fascinating world of commonsense reasoning in ai and its impact on the development of intelligent agents.
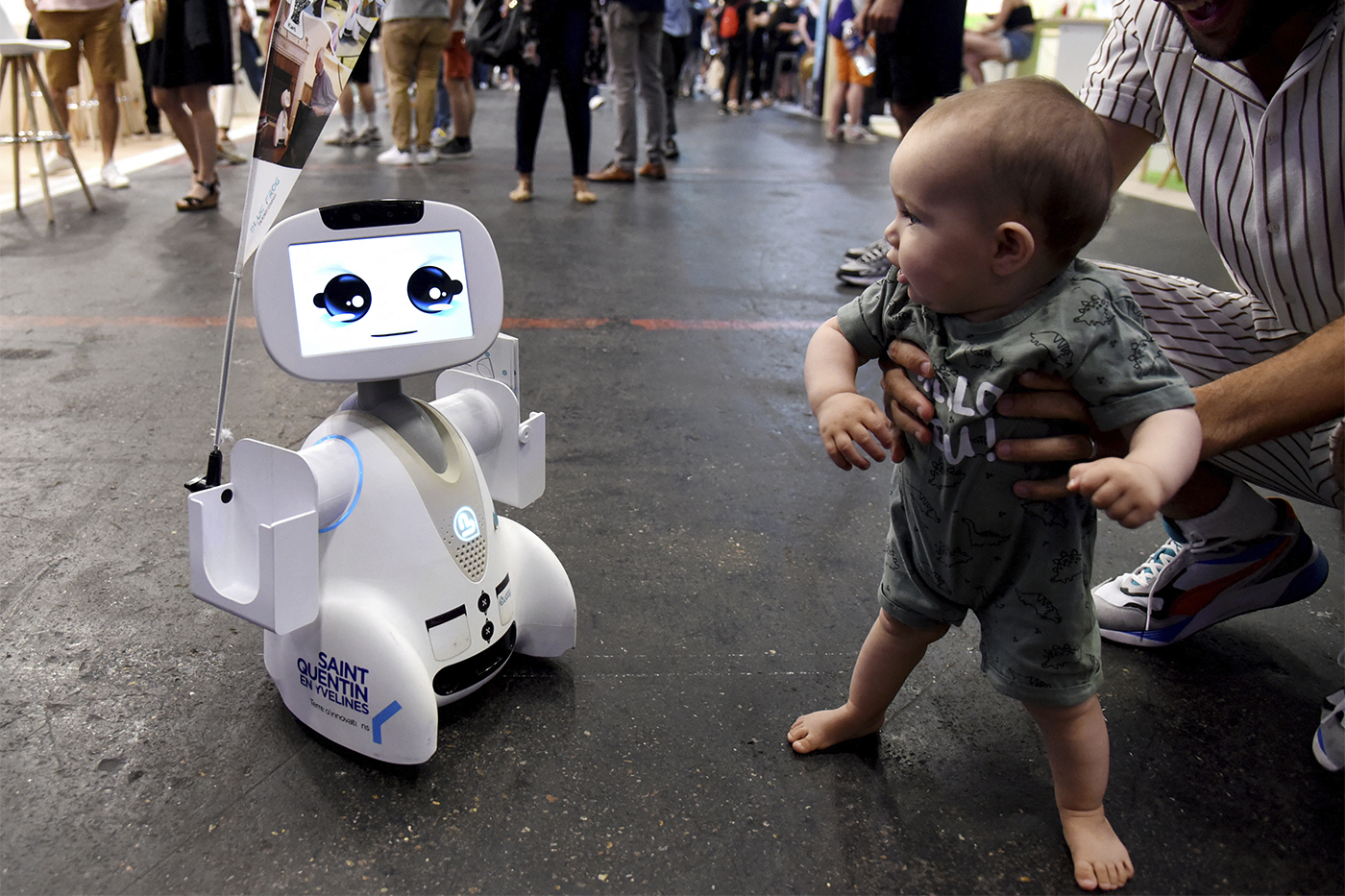
Credit: news.northeastern.edu
Understanding The Potential Of Commonsense Reasoning In Ai
The Importance Of Teaching Ai Agents About The World
Teaching ai agents about the world is crucial to enhance their capabilities and make them more intelligent. By incorporating commonsense reasoning into ai systems, we can equip them with a deeper understanding of the world around us. Here’s why teaching ai agents about the world is of utmost importance:
- Improved decision-making: Ai agents that possess commonsense reasoning abilities are better equipped to make informed decisions. They can consider a wider range of factors and draw conclusions based on their understanding of how the world works.
- Real-world problem-solving: Ai systems that lack commonsense reasoning often struggle to tackle real-world problems effectively. Teaching them about the world enables them to reason through scenarios and adapt their knowledge to new situations.
- Human-like interactions: Ai agents that comprehend commonsense knowledge can engage in more natural and intuitive conversations with humans. They can understand implicit meanings, interpret context, and actively participate in meaningful interactions.
- Reduced reliance on explicit data: While ai algorithms heavily rely on data, commonsense reasoning helps reduce the dependency on explicit data for every task. By understanding the world, ai agents can fill in the gaps and make inferences even when specific data might not be available.
- Enhanced creativity: Incorporating commonsense reasoning in ai opens up possibilities for creative problem-solving. Ai agents can analyze situations from various perspectives, explore unconventional solutions, and generate novel ideas based on their understanding of the world.
How Commonsense Reasoning Can Enhance Ai Capabilities
Commonsense reasoning can significantly enhance the capabilities of ai agents, enabling them to operate at an unprecedented level of intelligence. Here’s how commonsense reasoning can augment ai:
- Enhanced comprehension: Ai agents can understand and interpret natural language more accurately by incorporating commonsense reasoning. They can comprehend context, make sense of ambiguous statements, and grasp the implied meanings hidden within conversations.
- Contextual decision-making: With commonsense reasoning, ai agents can make decisions based on the prevailing context and situational understanding. This ability allows them to consider factors beyond the presented information and generate more contextually appropriate responses.
- Reasoning through uncertainty: Commonsense reasoning equips ai agents to handle uncertain and ambiguous scenarios effectively. They can leverage their knowledge about the world to reason through incomplete or conflicting information, leading to more accurate and reliable outcomes.
- Adaptive learning: Ai systems that incorporate commonsense reasoning can adapt and learn from new information more efficiently. They can generalize from specific instances to understand broader concepts and apply their knowledge to diverse situations.
- Ethical decision-making: Commonsense reasoning enables ai agents to make ethical judgments by understanding societal norms, values, and implicit rules. This capability is crucial in addressing ethical dilemmas and ensuring responsible and unbiased ai decision-making.
Overall, commonsense reasoning is a vital component in ai development. By teaching ai agents about the world, we empower them to comprehend, reason, and interact with humans in a more intuitive and intelligent manner.
Limited Data And Narrow Contexts
In the world of ai, common sense reasoning is crucial for training agents to understand and interact with the real world effectively. However, one of the significant challenges in this field is dealing with limited data and narrow contexts. Let’s explore the impact of these limitations in ai training and how they affect the understanding of real-world situations.
Data Limitations In Ai Training:
- Insufficient data: Many ai models require large amounts of data to train effectively. However, when it comes to common sense reasoning, obtaining sufficient data becomes a challenging task. The lack of diverse and comprehensive data can hinder the agent’s ability to generalize and make accurate predictions.
- Noisy data: Ai models rely on clean and reliable data for training. But in the case of commonsense reasoning, data can often be noisy or ambiguous. This can lead to incorrect or unpredictable outcomes, limiting the agent’s understanding of real-world scenarios.
- Bias in data: Data used for training ai models can inadvertently contain biases, reflecting the biases present in the human knowledge it learns from. This can result in skewed decision-making and reinforce societal biases. It is important to address and minimize these biases to ensure fair and unbiased reasoning.
Impact On Understanding Real-World Situations:
- Lack of context: Limited data and narrow contexts restrict an ai agent’s ability to understand complex real-world situations. This can make it challenging for agents to grasp the nuances and subtleties that humans effortlessly comprehend. Without a broader context, agents may struggle to make accurate inferences and provide meaningful responses.
- Difficulty in handling ambiguity: Real-world scenarios often involve ambiguous or uncertain information, which humans can navigate using their common sense. However, ai models constrained by limited data and narrow contexts find it hard to handle ambiguity. This limitation can hinder their ability to make informed decisions and may result in erroneous or inadequate responses.
- Inability to bridge gaps: Ai models may struggle to bridge gaps between different domains or understand connections that are not explicitly stated in the training data. This limits their capacity to transfer knowledge across various tasks and generalize from one context to another. Without this ability, agents may lack a comprehensive understanding of the world.
While limited data and narrow contexts pose significant challenges in teaching ai agents about the world, researchers are continuously exploring innovative approaches to address these limitations. By leveraging techniques such as transfer learning, pre-training, and knowledge graph construction, we can guide agents towards better sense-making abilities and equip them to handle diverse real-world situations more effectively.
As ai technology advances, we can expect these limitations to be lessened, leading to smarter, more context-aware agents capable of reasoning with increased sophistication.
Remember, understanding the limitations and challenges of data in ai training is crucial for building more robust and reliable ai systems with enhanced common sense reasoning abilities.
Ambiguity And Contextual Understanding
Dealing With Ambiguous Language And Contextual Nuances
Ambiguity is a common challenge in natural language processing (nlp) and artificial intelligence (ai). When it comes to training ai models, it’s important to teach them how to understand and interpret language that may have multiple meanings or relies heavily on contextual information.
Enhancing language models for better comprehension is key to addressing this issue. Here are a few key points to consider:
- Improving semantic understanding: Ai models need to be able to accurately interpret the meaning of words and sentences in various contexts. This can be achieved through techniques such as word embeddings, where words are represented by dense vectors that capture their semantic relationships.
- Leveraging contextual information: Context plays a vital role in disambiguating language. Ai models can be trained to consider the surrounding words and phrases to derive the intended meaning. Contextual embeddings, such as those used in models like bert (bidirectional encoder representations from transformers), help capture the broader context and improve the understanding of ambiguous language.
- Incorporating world knowledge: Teaching ai agents about the world can enhance their comprehension abilities. By providing them with a broad set of knowledge and facts, they can make more informed decisions and better understand the context in which language is used. Knowledge graphs and pre-training on large-scale datasets are some methods used to incorporate world knowledge into ai models.
- Domain-specific training: Different domains have their own unique language and terminology. Training language models on specific domains can help them develop a better understanding of the nuances and contextually specific language used within those domains. This domain-specific training can be particularly useful in areas like medical or legal fields, where precise and accurate language interpretation is crucial.
- Iterative learning: Ai models can benefit from iterative learning approaches, where they are trained on progressively more challenging and diverse datasets. This iterative process helps expose them to a wide range of language variations and ambiguity, further improving their ability to handle real-world scenarios.
- Human-in-the-loop approach: While ai models have made significant advancements in understanding language, human input is still valuable. Employing a human-in-the-loop approach, where human reviewers provide feedback and annotations, can help refine and continuously improve the language comprehension capabilities of ai models.
: embracing ambiguity and contextual nuances in language is crucial for training advanced ai models. By enhancing semantic understanding, leveraging contextual information, incorporating world knowledge, domain-specific training, iterative learning, and embracing human input, we can teach ai agents to navigate through ambiguous language and understand the subtleties of the world they are meant to navigate.
Ontologies And Knowledge Graphs
Building structured knowledge representation:
- One of the fundamental components of commonsense reasoning in ai is the ability to represent knowledge in a structured manner.
- Structured knowledge representations allow ai agents to understand and reason about the world more effectively.
- Ontologies and knowledge graphs are powerful tools for building such structured representations.
Leveraging ontologies for smarter reasoning:
- Ontologies are formal models that represent a domain’s concepts, properties, and relationships.
- They provide a shared understanding of a specific domain, enabling ai agents to reason and communicate effectively.
- By leveraging ontologies, ai agents can infer information that is not explicitly stated, making their reasoning smarter and more contextual.
- Ontologies also help in resolving ambiguity and identifying inconsistencies in a knowledge base.
Benefits of using knowledge graphs:
- Knowledge graphs are a form of knowledge representation that leverages ontologies to organize information in a graph-like structure.
- They interconnect concepts and entities, providing a rich contextual understanding of the relationships between them.
- Knowledge graphs enable ai agents to traverse and explore the interconnected knowledge, enhancing their reasoning capabilities.
- They also allow for the integration of diverse sources of information, facilitating a comprehensive understanding of the world.
Semantic querying and inference:
- Ontologies and knowledge graphs enable semantic querying, where ai agents can express complex queries using domain-specific terms.
- Semantic querying allows for precise and targeted retrieval of information, reducing ambiguity and improving efficiency.
- In addition to querying, ai agents can also perform inference on knowledge graphs to derive new insights and make more informed decisions.
- The ability to perform semantic querying and inference makes ai agents more intelligent and capable of understanding the complexities of the world.
Ontologies and knowledge graphs are essential tools for teaching ai agents about the world. They provide structured knowledge representations, enable smarter reasoning, and facilitate semantic querying and inference. By leveraging these techniques, ai agents can gain a deeper understanding of the world and make informed decisions based on contextual information.
Semantic Parsing And Natural Language Understanding
Converting Unstructured Data Into Structured Formats
Converting unstructured data into structured formats is a crucial step in teaching ai agents about the world. By structuring the data, we enable machine learning models to effectively extract meaning and make informed decisions. Here are some key points to consider:
- Unstructured data refers to information that lacks a predefined format, such as text documents, emails, or social media posts.
- Structured data, on the other hand, is organized and categorized, often represented in databases or spreadsheets.
- By converting unstructured data into structured formats, we can unlock the potential for ai agents to analyze and understand the information more efficiently.
- This process involves methods like data normalization, where information is standardized and transformed into a consistent format.
- Structuring data helps ai agents recognize patterns, relationships, and insights that might otherwise be difficult to identify in unstructured form.
Extracting Meaning From Natural Language Inputs
Extracting meaning from natural language inputs is a fundamental aspect of teaching ai agents to understand the world. Here are some key points to consider:
- Natural language understanding (nlu) focuses on the capability of ai agents to comprehend and interpret human language.
- Semantic parsing plays a crucial role in nlu by translating natural language inputs into machine-understandable representations.
- Ai models utilize semantic parsing techniques to extract meaning from sentences and map them to specific actions or responses.
- This process involves analyzing the syntax, grammar, and semantics of the input text to derive its intended meaning.
- Nlu enables ai agents to understand complex instructions, queries, or commands provided by humans, improving their ability to respond appropriately.
By harnessing techniques such as converting unstructured data into structured formats and extracting meaning from natural language inputs, we can enhance the commonsense reasoning of ai agents. These advancements contribute to their ability to understand the world and interact intelligently with users, ultimately leading to more efficient and effective ai-powered solutions.
Deep Learning And Commonsense Reasoning
Deep learning techniques have revolutionized many areas of artificial intelligence (ai), but when it comes to commonsense reasoning, they face significant challenges. Integrating deep learning methods with commonsense reasoning is a crucial step towards improving ai agents’ understanding of the world.
By training ai agents using large-scale datasets, we can enhance their ability to reason and make intelligent decisions. In this section, we will explore the key aspects of integrating deep learning techniques with commonsense reasoning.
Training Ai Agents Using Large-Scale Datasets
- Utilizing large-scale datasets provides ai agents with a broader knowledge base and improves their ability to understand the world.
- Training these agents with diverse and extensive data allows them to develop a more comprehensive understanding of human reasoning.
- By exposing ai agents to a vast array of scenarios and examples, we can help them acquire a thorough grasp of contextual knowledge and make more informed decisions.
Leveraging Deep Learning For Commonsense Reasoning
- Deep learning methods, such as neural networks, can process massive amounts of data and extract meaningful patterns, enabling ai agents to learn from diverse sources and derive valuable insights.
- These techniques enable ai agents to recognize and learn from complex relationships within the data, enhancing their ability to reason and make intelligent decisions.
- Deep learning models can be leveraged to extract structured information from unstructured data, allowing ai agents to grasp the implicit meaning and nuances present in commonsense reasoning tasks.
The Benefits Of Integrating Deep Learning With Commonsense Reasoning
- Combining deep learning with commonsense reasoning empowers ai agents to understand and interpret information more effectively.
- The integration of these techniques expands ai agents’ ability to reason by incorporating a broader scope of knowledge and learning from diverse examples.
- By incorporating commonsense reasoning into deep learning models, ai agents can make more accurate predictions and better address real-world challenges.
To summarize, integrating deep learning techniques with commonsense reasoning provides ai agents with the ability to understand the world and make informed decisions. By training these agents with large-scale datasets, we can enhance their understanding of contextual knowledge. Leveraging deep learning methods enables ai agents to extract valuable insights from diverse sources and uncover complex relationships.
The combination of these techniques unlocks the potential for ai agents to reason more effectively and tackle real-world problems.
Transfer Learning And Generalization Of Knowledge
Transfer learning, along with generalization of knowledge, is a crucial aspect of commonsense reasoning in ai. It enables agents to leverage their learned knowledge from one domain and apply it efficiently to another. This process facilitates the improvement of generalization, allowing ai systems to make informed decisions and predictions in unfamiliar situations.
Let’s explore how transfer learning and generalization play a significant role in teaching agents about the world.
Transferring Knowledge From One Domain To Another
Transferring knowledge from one domain to another involves reusing what an ai agent has learned in a specific context and applying it to a different but related domain. Here are the key points to consider:
- Previous learning: An ai agent begins by acquiring knowledge and expertise in a particular domain through supervised or unsupervised learning techniques.
- Initialization: After the agent has acquired foundational knowledge, it undergoes a transfer learning process, where the initial model is initialized using the pre-existing knowledge.
- Task adaptation: The agent then adapts its knowledge to the new domain, fine-tuning its model by considering the specific features and dynamics of the new dataset.
- Performance enhancement: By transferring knowledge, ai agents can enhance their performance in the new domain, leveraging the insights gained from previous experiences.
Improving Generalization Using Transfer Learning Approaches
Transfer learning approaches are instrumental in improving generalization capabilities in ai systems. Here are the key points to understand:
- Common patterns: Transfer learning helps agents identify common patterns and features across different domains, enabling them to recognize similarities and effectively generalize their knowledge.
- Domain adaptation: Through transfer learning, an agent learns to adapt its knowledge and reasoning skills from one domain to another, even if the datasets are not identical.
- Reduced data requirements: Transfer learning allows agents to learn efficiently from limited data, as they can leverage previously learned knowledge from related domains, mitigating the need for an extensive dataset in each new domain.
- Faster learning: By transferring knowledge, agents can learn faster in new domains, as they can build upon the understanding they have already developed, reducing the time and computational resources required for training.
Transfer learning and generalization of knowledge are pivotal in ai systems’ ability to reason and make sense of the world. By transferring knowledge from one domain to another, and leveraging transfer learning approaches, agents can enhance their ability to generalize and apply their learned knowledge effectively.
These advancements contribute to the development of ai systems that exhibit better decision-making, problem-solving, and predictive capabilities, bridging the gap between human-like commonsense reasoning and ai.
Frequently Asked Questions Of Commonsense Reasoning In Ai – Teaching Agents About The World
How Does Ai Learn Commonsense Reasoning?
Ai learns commonsense reasoning through machine learning algorithms that analyze large datasets to identify patterns and make inferences about the world.
Why Is Commonsense Reasoning Important For Ai?
Commonsense reasoning is crucial for ai as it helps agents understand the world, make informed decisions, and interact with humans in a more intelligent and natural way.
What Challenges Are There In Teaching Agents About The World?
Teaching agents about the world poses challenges like acquiring commonsense knowledge, understanding context, handling ambiguity, and correctly interpreting human instructions.
How Can Ai Benefit From Commonsense Reasoning?
By incorporating commonsense reasoning, ai can provide better recommendations, assist in problem-solving, enhance natural language understanding, and improve decision-making processes.
What Are The Real-World Applications Of Commonsense Reasoning In Ai?
Commonsense reasoning finds applications in areas like virtual assistants, autonomous vehicles, customer support, healthcare diagnosis, and smart home technology, to name a few.
Conclusion
The development of commonsense reasoning in ai has shown great promise in teaching agents about the world. By understanding the nuances and complexities of everyday situations, ai systems can make more informed decisions and perform tasks that were once thought to be exclusive to human intelligence.
Through advancements in natural language processing, machine learning, and knowledge representation, ai researchers are working towards equipping machines with a deeper understanding of context, causality, and common sense. This has significant implications for various industries, including healthcare, finance, and customer service, where machines can assist and complement human decision making.
As commonsense reasoning evolves, it is important to address ethical considerations and ensure that ai systems are aligned with human values and norms. Furthermore, continued research and collaboration among experts will be crucial in refining and expanding the capabilities of ai systems, ultimately leading to smarter, more intuitive machines that can navigate the complexities of the world with greater efficiency and accuracy.