Disentangling causal inference involves employing techniques to establish cause-effect relationships. We will explore various methods used for establishing cause-effect relationships, providing a comprehensive overview of their effectiveness and applications.
Understanding causal relationships is crucial in various fields, including social sciences, healthcare, and marketing. By identifying cause-effect relationships, we can make informed decisions, develop effective strategies, and implement targeted interventions. Throughout this article, we will delve into the different techniques used to determine causality, highlighting their strengths, limitations, and appropriate contexts for implementation.
Whether you are a researcher, policymaker, or business professional, this article will equip you with the knowledge and tools necessary for accurate causal inference. So, let’s dive in and unravel the complexities of cause-effect relationships.
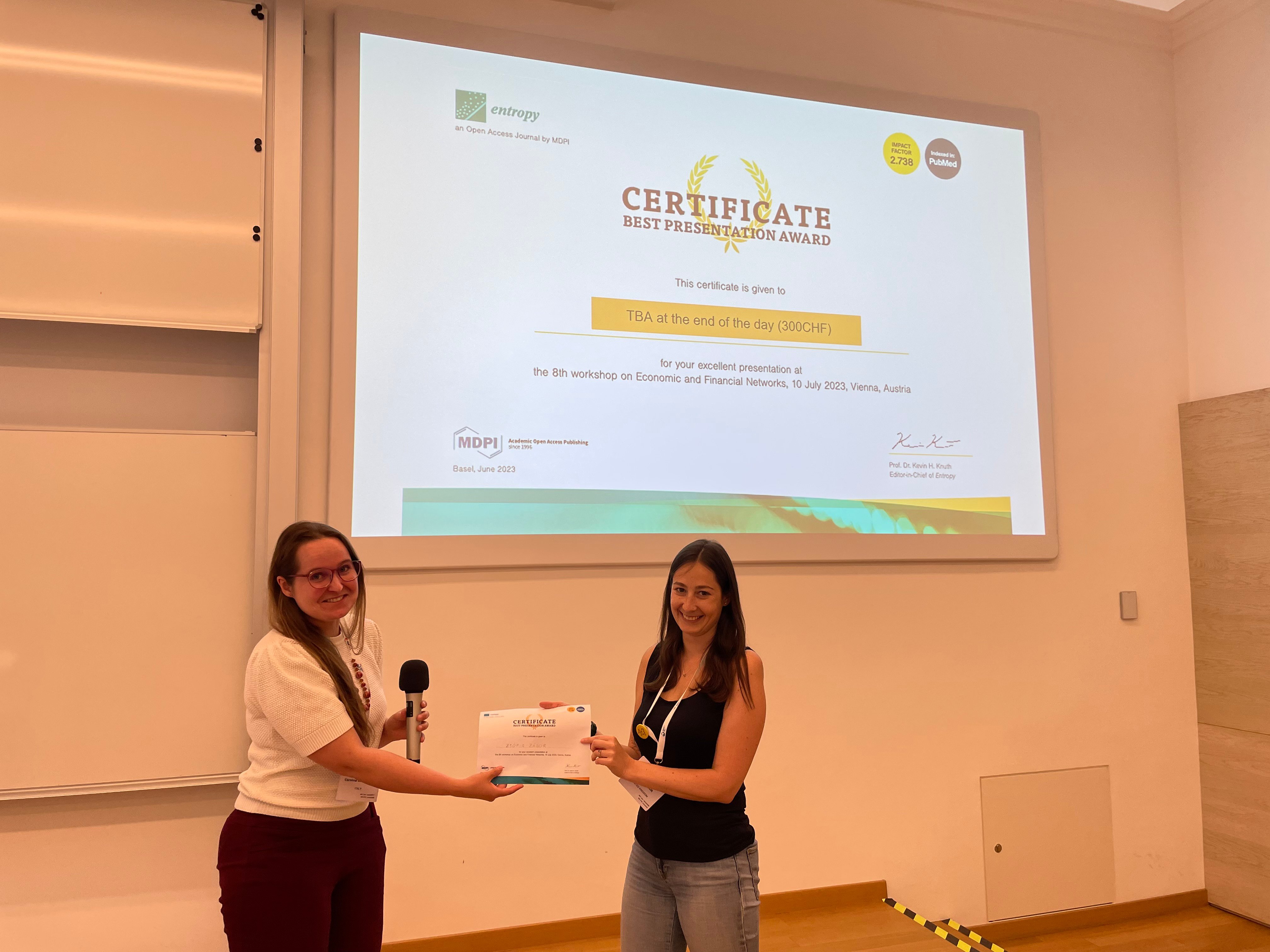
Credit: www.mdpi.com
Frequently Asked Questions For Disentangling Causal Inference – Techniques For Establishing Cause-Effect
Q: What Techniques Are Used For Establishing Cause-Effect Relationships?
A: different techniques such as randomized controlled trials, natural experiments, and instrumental variables can be used.
Q: How Can Randomized Controlled Trials Help Establish Causality?
A: randomized controlled trials assign participants randomly to treatment groups, eliminating confounding factors and allowing causal inferences.
Q: What Are Natural Experiments And How Do They Help Establish Cause-Effect Relationships?
A: natural experiments occur when treatment groups are formed naturally, offering researchers an opportunity to analyze cause-effect relationships in real-world settings.
Q: How Do Instrumental Variables Contribute To Causal Inference?
A: instrumental variables are used to estimate causal effects by isolating the variation in the treatment that is unrelated to confounding factors.
Q: Why Is It Important To Disentangle Causal Inference For Research Studies?
A: disentangling causal inference helps researchers understand the true impact of interventions, policies, or treatments, leading to more accurate conclusions and informed decision-making.
Conclusion
Establishing cause-effect relationships is a complex task that requires methodological rigor and strategic thinking. By disentangling causal inference techniques, we can unveil the true effect of an intervention or variable on an outcome, leading to more informed decision-making. Throughout this blog post, we have explored various approaches such as randomized control trials, natural experiments, and instrumental variables.
Each technique has its strengths and limitations, but they all provide valuable insights into the causal relationships under investigation. It is important to carefully select the most appropriate technique based on the research question and available data. Furthermore, understanding common pitfalls and biases associated with causal inference is crucial for obtaining accurate and reliable results.
As researchers and decision-makers, we must continually strive for improved methodologies and deeper understanding of causality. By doing so, we can contribute to a more evidence-based and impactful decision-making process, ultimately leading to positive societal changes.