Quantum computing powers ai by utilizing the unique properties of quantum systems, such as superposition and entanglement, to enhance machine learning algorithms. We will explore the intersection of quantum computing and ai, discussing how quantum machine learning algorithms are developed and how they are revolutionizing various industries with their potential for solving complex problems more efficiently.
We will also examine the challenges and limitations faced in implementing quantum machine learning, along with the future prospects of this exciting field. So, let’s dive into the world of quantum machine learning and discover the groundbreaking advancements it brings to the field of artificial intelligence.
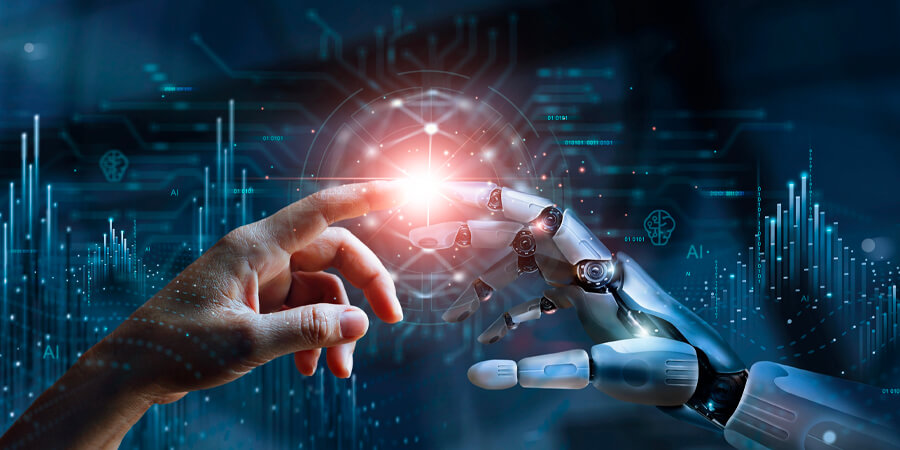
Credit: www.telecomreviewafrica.com
What Is Quantum Machine Learning?
Quantum Machine Learning – How Quantum Computing Powers Ai
Quantum computing and machine learning are two cutting-edge fields that are revolutionizing technology as we know it. Individually, they hold immense potential, but when combined, they become a force to be reckoned with. In this section, we will delve into the world of quantum machine learning, exploring the intersection of quantum computing and machine learning, and how this fusion is driving the advancement of artificial intelligence (ai) to new heights.
Brief Explanation Of Quantum Computing And Machine Learning
Quantum computing:
- Quantum computing harnesses the principles of quantum mechanics to perform calculations at an extraordinary pace, surpassing the capabilities of classical computers.
- Instead of using traditional bits, quantum computers utilize quantum bits, or qubits, which can represent multiple states simultaneously, enabling parallel processing.
- Quantum computers are known for their ability to solve complex mathematical problems that would take classical computers an astronomical amount of time, making them particularly suitable for solving optimization and simulation tasks.
Machine learning:
- Machine learning is a subset of ai that empowers computer systems to learn and improve from experience without being explicitly programmed.
- It involves the development of algorithms and models that enable machines to learn patterns and make predictions or decisions based on data inputs.
- Machine learning algorithms can be broadly categorized into supervised learning, unsupervised learning, and reinforcement learning, depending on the presence or absence of labeled training data.
Showcasing The Intersection Of The Two Fields
The synergy between quantum computing and machine learning has opened up a world of possibilities for ai advancement. Here are some key points to highlight this intersection:
Quantum-enhanced optimization:
- Quantum computers excel in solving optimization problems, which are fundamental to various machine learning tasks.
- By leveraging the parallelism and computational power of qubits, quantum computing can significantly speed up optimization algorithms used in machine learning.
- Quantum algorithms like the quantum approximate optimization algorithm (qaoa) are being developed to enhance optimization tasks, with applications in fields such as portfolio optimization and logistics planning.
Quantum-inspired machine learning algorithms:
- Quantum machine learning algorithms leverage the principles of quantum physics to enhance classical machine learning techniques.
- These algorithms explore quantum-inspired feature mapping, kernel methods, and other strategies to optimize processing and efficiently extract patterns from complex datasets.
- Quantum-inspired machine learning holds promise for solving real-world problems, such as drug discovery, financial prediction, and natural language processing, with improved accuracy and efficiency.
Quantum data analysis and dimensionality reduction:
- The vast amounts of data generated in today’s digital world pose a challenge in terms of analysis and dimensionality reduction.
- Quantum computing can potentially overcome this challenge by efficiently processing large datasets and extracting meaningful insights.
- Quantum algorithms like quantum singular value transformation (qsvt) offer a quantum advantage in data analysis tasks, aiding the identification of hidden patterns and reducing dimensions for improved machine learning performance.
Quantum machine learning is an exciting field that combines the power of quantum computing with the intelligence of machine learning algorithms. This fusion holds immense potential for solving complex problems, accelerating optimization tasks, and advancing the capabilities of ai. As quantum technologies continue to evolve, we can expect quantum machine learning to unlock new frontiers in artificial intelligence and reshape various industries.
Advantages Of Quantum Machine Learning
Quantum Machine Learning – How Quantum Computing Powers Ai
Imagine a world where computers could process massive amounts of data simultaneously, leading to advancements in artificial intelligence (ai) that were once inconceivable. Quantum machine learning, the fusion of quantum computing and ai, has the potential to revolutionize industries and solve complex problems at an unprecedented pace.
In this section, we will delve into the advantages offered by quantum machine learning, shedding light on the remarkable capabilities that this cutting-edge technology provides.
Faster Processing Speed Due To Quantum Parallelism
Quantum machine learning harnesses the power of quantum parallelism to execute tasks at remarkable speeds. Traditional computers process information sequentially, meaning they tackle one problem at a time. On the other hand, quantum computers exploit quantum bits, or qubits, which can exist in a superposition of both 0 and 1 simultaneously.
This allows for parallel computation, enabling quantum machine learning algorithms to process vast amounts of data concurrently, accelerating computation exponentially.
Bullet points:
- Quantum parallelism enables simultaneous processing of multiple paths, vastly enhancing the speed of computations.
- The ability to explore various possibilities concurrently reduces the time required to achieve results.
- Quantum machine learning leverages the computational abilities of qubits to solve complex problems faster than classical computing methods.
Improved Accuracy And Efficiency In Data Analysis
Quantum machine learning presents unparalleled accuracy and efficiency in analyzing vast quantities of data. Traditional machine learning algorithms often struggle with large datasets, resulting in delays and compromised accuracy. Quantum machine learning algorithms, however, harness the power of quantum computing to perform complex calculations with greater precision and efficiency.
Bullet points:
- Quantum machine learning algorithms excel in analyzing complex datasets, enabling more accurate predictions and insights.
- Quantum algorithms leverage quantum states to efficiently process and analyze data, leading to improved accuracy.
- By utilizing quantum principles, quantum machine learning reduces the chances of errors and noise interference, enhancing the overall efficiency of data analysis.
Quantum machine learning offers significant advantages over traditional computing methods. Its ability to leverage quantum parallelism results in faster processing speeds, opening pathways to solving complex problems in a fraction of the time. Additionally, the improved accuracy and efficiency in data analysis enable more precise predictions and insights.
As this innovative field continues to evolve, the intersection of quantum computing and ai presents innumerable possibilities for advancements in various industries.
Quantum Machine Learning In Drug Discovery
————————————————-
The use of quantum computing in the field of drug discovery is a groundbreaking application of this emerging technology. By leveraging quantum algorithms, researchers are able to accelerate the process of finding new drugs and improve precision in molecular modeling and virtual screening.
Let’s delve into the details of how quantum machine learning is revolutionizing drug discovery.
Accelerating The Process Of Finding New Drugs Using Quantum Algorithms:
- Quantum algorithms offer the potential to significantly speed up the drug discovery process by harnessing the power of quantum computing.
- Quantum machine learning algorithms can analyze massive amounts of data and perform complex calculations exponentially faster than classical computers.
- By quickly generating and analyzing vast amounts of chemical data, quantum algorithms can help researchers identify potential drug candidates more efficiently.
- Quantum computers can also simulate and predict the behavior of molecules with greater accuracy, providing valuable insights into their interactions and aiding the development of new drugs.
Improving Precision In Molecular Modeling And Virtual Screening:
- Quantum machine learning enables more accurate molecular modeling, allowing researchers to better understand the behavior and properties of complex molecules.
- Quantum computers can simulate molecular structures with exceptional precision, providing a deeper understanding of how different compounds interact at the atomic level.
- By incorporating quantum algorithms into virtual screening techniques, scientists can identify potential drug candidates that exhibit specific properties or target specific biomarkers.
- These advancements in precision enable researchers to design drugs with enhanced efficacy and fewer side effects, potentially revolutionizing the field of medicine.
Quantum machine learning is transforming the field of drug discovery by accelerating the process of finding new drugs and improving precision in molecular modeling and virtual screening. This powerful combination of quantum computing and artificial intelligence has the potential to reshape the pharmaceutical industry and bring about new advancements in healthcare.
Quantum Machine Learning In Finance
Utilizing Quantum Algorithms For Predicting Financial Trends
The emerging field of quantum machine learning has the potential to revolutionize the world of finance. By harnessing the power of quantum computing, financial institutions can enhance their prediction capabilities and make more accurate forecasts of financial trends. Here are the key points:
- Quantum algorithms offer a significant advantage over classical algorithms in terms of processing power. This enables financial analysts to handle complex datasets and perform intricate calculations that were previously impossible.
- Quantum machine learning algorithms can extract hidden patterns and correlations from financial data, allowing for more accurate predictions of market trends. This can help investors make informed decisions and potentially maximize their profits.
- Quantum computers have the ability to process multiple possibilities simultaneously through quantum superposition. This enables the exploration of various scenarios and outcomes, providing a more comprehensive view of market dynamics.
- The quantum nature of these algorithms also allows for enhanced speed and efficiency. This can greatly reduce the time required for data analysis and enable real-time decision-making.
- By leveraging quantum machine learning, financial institutions can gain a competitive edge by staying ahead of market fluctuations and adapting their investment strategies accordingly.
Enhancing Risk Assessment And Portfolio Optimization Strategies
In addition to predicting financial trends, quantum machine learning can also enhance risk assessment and portfolio optimization strategies. Here’s how:
- Quantum algorithms can efficiently handle large and complex datasets, enabling a more detailed analysis of risk factors. This can help financial institutions assess and mitigate potential risks more effectively.
- Quantum machine learning can provide a more holistic view of risk by considering various variables simultaneously. By incorporating multiple factors, such as market conditions, economic indicators, and historical data, the accuracy of risk assessment models can be significantly improved.
- Portfolio optimization is another area where quantum machine learning can make a substantial impact. With the ability to process vast amounts of data and analyze correlations, quantum algorithms can identify optimal investment combinations and asset allocations.
- This can lead to more balanced and diversified portfolios, reducing the risk of losses and increasing the potential for higher returns.
- Moreover, quantum machine learning can help financial institutions identify and react to changing market conditions in real-time. By continuously monitoring the financial ecosystem, adjustments can be made promptly, ensuring optimal portfolio performance.
Quantum machine learning in finance offers exciting opportunities for predicting financial trends, enhancing risk assessment, and optimizing portfolios. By harnessing the power of quantum computing, financial institutions can make more accurate predictions and gain a competitive edge in the financial landscape.
It’s clear that the integration of quantum machine learning into the realm of finance has the potential to reshape the industry as we know it.
Quantum Machine Learning In Image Recognition
———————————————-
With the rapid advancements in technology, machine learning has become an integral part of our lives. From voice assistants to recommendation algorithms, it has revolutionized the way we interact with machines. But what if we could take machine learning to the next level?
That’s exactly where quantum machine learning comes into play. By harnessing the power of quantum computing, we can unlock new possibilities in ai, particularly in the field of image recognition.
Enhancing Image Recognition Capabilities Through Quantum Neural Networks
Quantum machine learning has the potential to greatly enhance image recognition capabilities. Here are a few key points to understand how quantum neural networks can revolutionize image recognition:
- Quantum neural networks leverage the principles of quantum mechanics to process and analyze vast amounts of data simultaneously. This parallel processing power allows for more complex computations and enables the handling of large-scale image recognition tasks with improved efficiency.
- One of the key advantages of quantum machine learning in image recognition is its ability to work with high-dimensional data. Traditional machine learning algorithms often struggle with the complexity of image data, but quantum neural networks can handle these challenges more effectively and extract meaningful features from images.
- Quantum machine learning can improve the accuracy of object identification and classification. By utilizing the unique properties of quantum computing, these algorithms can uncover intricate patterns and subtle details in images, leading to more precise and reliable results. This can have numerous applications in various fields, from medical imaging to autonomous vehicles.
- Another area where quantum machine learning shines in image recognition is in handling noisy or incomplete data. Traditional machine learning models often struggle when faced with imperfect or corrupted images, but quantum algorithms can effectively deal with these issues, enabling better recognition and classification even in challenging scenarios.
Improving Object Identification And Classification Accuracy
Quantum machine learning has the potential to significantly improve the accuracy of object identification and classification tasks. Here’s a brief overview of how it achieves this:
- Quantum algorithms for image recognition can leverage quantum superposition and entanglement to process multiple possibilities simultaneously. This allows for a more comprehensive analysis of the images and enables the identification of subtle patterns that might be missed by classical algorithms.
- Quantum machine learning algorithms also have the advantage of being highly adaptive and trainable. They can continuously learn and adjust their models based on new data, leading to improved accuracy over time. This adaptability is particularly useful in dynamic environments where the characteristics of objects may change or evolve.
- With the ability to handle high-dimensional data and complex computations, quantum machine learning algorithms can tackle more challenging image recognition tasks, such as detecting objects in cluttered scenes or recognizing fine-grained details. This can have practical applications in fields like surveillance, robotics, and quality control.
- The combination of quantum computing and machine learning in image recognition opens up new avenues for innovation and research. Scientists and researchers are actively exploring ways to optimize quantum algorithms for specific image recognition tasks and to harness the full power of quantum computing in this field.
Quantum machine learning has the potential to revolutionize image recognition by enhancing capabilities, improving accuracy, and expanding the applications of this technology. As we continue to unlock the power of quantum computing, we can expect further advancements in ai and image recognition that will shape the future of various industries.
Overcoming Quantum Noise And Error Correction
Quantum Machine Learning – How Quantum Computing Powers Ai
Quantum computing has emerged as a revolutionary field that holds the potential to transform various industries, including artificial intelligence (ai). As researchers explore the integration of quantum mechanics with machine learning principles, they have encountered significant challenges, particularly related to quantum noise and error correction.
In this section, we will delve into strategies for reducing noise and improving qubit reliability, as well as explore error correction techniques in quantum machine learning.
Strategies For Reducing Noise And Improving Qubit Reliability
- Quantum noise, arising from external factors and imperfect control mechanisms, poses a fundamental challenge to the reliability of qubits. However, several strategies have been developed to mitigate this issue:
- Interleaved randomized benchmarking: This technique involves interleaving quantum gates with randomized benchmarking sequences to evaluate and quantify the noise impacting a qubit. By characterizing and understanding the noise, researchers can devise strategies to mitigate its effects.
- Dynamical decoupling: This method involves applying control pulses to qubits at regular intervals to mitigate the impact of external noise. By continuously refreshing the state of the qubits, researchers aim to prolong coherence times and improve overall qubit reliability.
- Quantum error correction: Quantum error correction codes provide a way to detect and correct errors caused by noise. These codes make use of additional qubits known as ancilla qubits to encode information redundantly, thereby protecting it against the effects of noise.
Exploration Of Error Correction Techniques In Quantum Machine Learning
- Error correction techniques play a crucial role in addressing noise-induced errors in quantum machine learning models. Here are some key approaches:
- Surface code: One popular error correction code is the surface code. It utilizes a two-dimensional layout of qubits, where qubits in the center act as data qubits, while qubits around the edges serve as ancilla qubits. By monitoring and correcting errors on the surface through stabilizer measurements, the surface code enhances the reliability of quantum computations.
- Topological codes: These codes make use of nontrivial topological properties to protect qubits from errors. By exploiting the concept of anyons, which are fractionalized quantum excitations carrying quantum information, topological codes provide an efficient means of error detection and correction.
- Concatenated codes: Concatenated codes involve cascading multiple layers of error correction codes to enhance the overall fault-tolerance of quantum systems. By repeatedly applying error correction at various levels, researchers can improve the stability and reliability of quantum computations.
Overcoming quantum noise and implementing effective error correction techniques are crucial steps towards harnessing the power of quantum computing in ai. Strategies such as interleaved randomized benchmarking and dynamical decoupling help in reducing noise and enhancing qubit reliability, while error correction techniques like the surface code, topological codes, and concatenated codes ensure the accuracy of quantum machine learning models.
As researchers continue to advance in this space, quantum machine learning holds immense promise for driving breakthroughs in artificial intelligence and shaping the future of technology.
Advancements In Quantum Machine Learning Hardware
Quantum Machine Learning – How Quantum Computing Powers Ai
Quantum computing has emerged as a revolutionary technology with the potential to transform various fields, including machine learning. With its ability to process vast amounts of data and perform complex calculations at an unprecedented speed, quantum computing offers exciting possibilities for advancing artificial intelligence.
In this section, we will explore the advancements in quantum machine learning hardware and their impact on the field.
Overview Of Quantum Hardware Advancements And Their Impact On Machine Learning
The development of quantum hardware has been critical in harnessing the power of quantum computing for machine learning applications. Here are some key points to consider:
- Increased qubit count: Quantum machine learning requires a large number of qubits to process and manipulate data effectively. Recent advancements have witnessed an increase in qubit counts, with quantum systems now capable of reaching 50 qubits or more. This increase in qubit count opens up new possibilities for complex computational tasks and enables more robust machine learning algorithms.
- Improved coherence times: Coherence time refers to the period during which qubits can maintain their quantum state without significant degradation. Longer coherence times are crucial for accurate computation and error correction in quantum machine learning. Recent hardware advancements have extended the coherence times of qubits, allowing for more precise and reliable calculations.
- Enhanced gate operations: The ability to perform gate operations with high fidelity is crucial for quantum computing. Advancements in quantum hardware have led to improved gate fidelities, enabling more accurate manipulation of qubits and reducing errors in quantum circuits. This enhancement in gate operations paves the way for more efficient and reliable training of machine learning models.
- Quantum error correction: Quantum systems are susceptible to errors caused by environmental factors and noise. Quantum error correction techniques mitigate these errors, ensuring the accuracy and reliability of quantum computations. Hardware advancements have facilitated the implementation of error correction codes, making quantum machine learning systems more resilient to errors and improving the overall performance of ai algorithms.
Potential For Scalable And Practical Quantum Machine Learning Systems
The advancements in quantum hardware hold tremendous potential for the development of scalable and practical quantum machine learning systems. Consider the following points:
- Faster training of machine learning models: Quantum computing’s immense computational power allows for the rapid training of complex machine learning models. Quantum algorithms, such as the quantum support vector machine and quantum neural networks, can process and analyze data more efficiently, reducing training time and enhancing model performance.
- Solving optimization problems: Optimization lies at the core of many machine learning tasks, and quantum computing excels in solving optimization problems through methods like quantum annealing and quantum-inspired algorithms. These techniques can optimize parameters, identify patterns, and improve decision-making processes in machine learning.
- Enhanced pattern recognition: Quantum machine learning algorithms have the potential to excel in pattern recognition tasks, such as image and speech recognition. The increased computational capabilities of quantum hardware enable more intricate analysis of data, leading to improved accuracy and robustness in pattern recognition applications.
- Quantum data processing: Quantum computing’s ability to process vast amounts of data in parallel opens up new avenues for big data processing and analysis. Quantum machine learning systems can handle large datasets more efficiently, allowing for deeper insights and more comprehensive analysis of complex data structures.
As advancements in quantum hardware continue to unfold, we can expect even greater breakthroughs in the field of quantum machine learning. These advancements will not only augment ai capabilities but also pave the way for the development of innovative applications across various domains.
By harnessing the power of quantum computing, we are forging ahead into an era of unprecedented possibilities for artificial intelligence.
Ethical Considerations In Quantum Machine Learning
Quantum Machine Learning – How Quantum Computing Powers Ai
Quantum machine learning is an emerging field that combines the power of quantum computing with artificial intelligence (ai) algorithms. As this technology continues to evolve, it is essential to consider the ethical implications it brings forth. In this section, we will discuss two key ethical considerations in quantum machine learning: privacy and security, and addressing biases and transparency in algorithms for fair decision-making.
Discussion On The Implications Of Quantum Machine Learning On Privacy And Security
- Privacy concerns:
- Quantum machine learning algorithms can process vast amounts of data, which can include sensitive personal information. Ensuring the privacy and protection of this data becomes crucial to maintain trust.
- The use of quantum computers to break current encryption methods poses a potential threat to data privacy. New encryption techniques need to be developed to secure sensitive information effectively.
- Quantum machine learning may raise concerns about data ownership and control. Establishing clear guidelines and regulations on data usage and ownership becomes paramount.
- Security challenges:
- Quantum computers have the potential to solve computational problems much faster than classical computers, including breaking encryption algorithms. This poses a significant security risk to existing security protocols.
- Developing quantum-resistant encryption algorithms is essential to safeguard data against potential cyber threats.
- Quantum machine learning algorithms can be vulnerable to adversarial attacks, where malicious actors manipulate data inputs to influence algorithmic decision-making. Robust security measures should be put in place to detect and mitigate such attacks effectively.
Addressing Biases And Transparency In Algorithms For Fair Decision-Making
- Bias in quantum machine learning:
- Just like classical machine learning, quantum machine learning algorithms can be susceptible to biases present in the training data. Bias could lead to unfair or discriminatory outcomes in decision-making processes.
- It is crucial to identify and mitigate biases in the data used for training quantum machine learning models. Ensuring diversity and inclusiveness in the dataset is a crucial step towards reducing bias.
- Transparency in algorithmic decision-making:
- Quantum machine learning algorithms are often complex, making it challenging to understand the factors that contribute to their decisions. Lack of transparency can hinder accountability and ethical decision-making.
- Efforts should be made to develop transparent algorithms that provide explanations for their decision outputs. This would enable users to understand the reasoning behind the decisions made by quantum machine learning models.
- Promoting transparency in quantum machine learning algorithms fosters trust and ensures fair decision-making processes.
As quantum machine learning continues to advance, it is essential to address the ethical considerations surrounding privacy, security, bias, and transparency. By prioritizing these concerns, we can harness the potential of quantum computing and artificial intelligence in a responsible and equitable manner, benefiting both individuals and society as a whole.
Frequently Asked Questions Of Quantum Machine Learning – How Quantum Computing Powers Ai
What Is Quantum Machine Learning?
Quantum machine learning is the intersection of quantum computing and artificial intelligence to enhance data analysis and improve machine learning algorithms.
How Does Quantum Computing Power Ai?
Quantum computing leverages the principles of quantum mechanics to process vast amounts of data simultaneously, accelerating ai algorithms and solving complex problems efficiently.
Can Quantum Machine Learning Outperform Classical Machine Learning?
Yes, quantum machine learning has the potential to outperform classical machine learning by handling more complicated calculations and modeling more extensive datasets.
What Are The Advantages Of Quantum Machine Learning Over Classical Machine Learning?
Quantum machine learning offers significant advantages such as faster processing, improved pattern recognition, enhanced optimization capabilities, and the ability to solve complex problems more effectively.
How Can Quantum Machine Learning Impact Various Industries?
Quantum machine learning can revolutionize industries like drug discovery, finance, logistics, and cybersecurity by enabling faster and more accurate predictions, optimization, and data analysis.
Conclusion
Quantum machine learning holds immense potential to revolutionize the field of ai by harnessing the power of quantum computing. As we have explored in this blog post, quantum computers can exponentially speed up complex computations, allowing for more efficient and accurate machine learning algorithms.
By taking advantage of phenomena like superposition and entanglement, quantum machine learning opens up new possibilities in areas such as drug discovery, optimization problems, and data analysis. Despite the current limitations and challenges in developing practical quantum machine learning algorithms, research in this field is rapidly progressing.
As quantum computing continues to advance, the integration of quantum machine learning into various industries will undoubtedly lead to transformative breakthroughs. It is an exciting time to be in the realm of artificial intelligence, and as we move forward, we can expect to witness further advancements and innovative applications of quantum machine learning.