Reality vs hype – a balanced look at large language models like gpt-3: large language models like gpt-3 are highly advanced ai technology capable of generating human-like text, but understanding the reality behind their capabilities is important to avoid falling prey to the hype. These models have shown impressive results in various domains, but they also have limitations and ethical concerns to consider.
We will delve into the actual capabilities of gpt-3, separating fact from fiction and providing a well-rounded understanding of its potential and limitations.
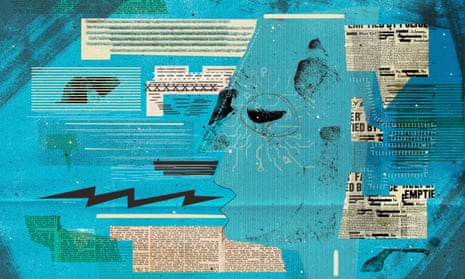
Credit: www.theguardian.com
Myth 1: Gpt-3 Will Replace Human Writers And Content Creators
Artificial intelligence (ai) has made remarkable advancements in recent years, and one of the most buzzed-about developments is gpt-3, a large language model. However, there is some hype surrounding gpt-3 that needs to be addressed. Let’s examine the reality behind one of the most common misconceptions: gpt-3 will replace human writers and content creators.
Examining The Limitations And Biases Of Gpt-3
Gpt-3 is undoubtedly an impressive creation, capable of generating coherent and contextually relevant text. However, it is crucial to understand its limitations and potential biases before dismissing the importance of human writers and content creators. Consider the following points:
- Gpt-3’s responses are generated based on patterns and information it has learned from the vast amount of data it has been trained on. While it can produce coherent text, it lacks a deep understanding of the nuances, emotions, and intent that human writers bring to their work.
- Gpt-3 may generate text that sounds plausible but is factually incorrect. It is not equipped with the ability to verify information or draw upon personal experiences and expertise like human writers can. Consequently, it may inadvertently produce inaccurate or misleading content.
- Bias is an inherent challenge when working with ai models like gpt-3. The biases present in training data can seep into the generated content. Although efforts are made to mitigate biases, complete elimination is challenging. Human writers, on the other hand, are capable of consciously addressing biases and providing diverse perspectives to ensure balanced and fair content.
- Gpt-3 lacks creativity and the ability to think critically. It cannot come up with novel ideas or concepts on its own. Human writers possess the unique ability to think outside the box, draw connections, and provide fresh insights that captivate and engage readers.
The Importance Of Human Touch In Content Creation
While gpt-3 can be a helpful tool in certain situations, it cannot replace human writers and content creators entirely. Here’s why the human touch remains essential:
- Human writers offer a personal touch, infusing their content with their unique voice, creativity, and style. This personal touch adds depth and authenticity that captivates readers and forges meaningful connections.
- Human writers possess subject matter expertise, industry knowledge, and years of experience, enabling them to produce insightful, accurate, and valuable content. Their ability to conduct research, fact-check, and validate information is unmatched.
- Content creation goes beyond mere words on a page. It involves understanding the target audience, tailoring content to their needs, and deploying strategic thinking to create content that resonates and drives desired outcomes. Human writers excel in this aspect, using their emotional intelligence and intuition to craft persuasive and compelling content.
Although gpt-3 showcases impressive language generation capabilities, it should be seen as a tool to complement human writers rather than a direct replacement. The expertise, creativity, critical thinking, and human touch that writers bring to the table cannot be replicated by ai.
By leveraging the strengths of both ai and human writers, we can create content that is informative, engaging, and resonates deeply with readers.
Myth 2: Gpt-3 Possesses True Understanding And Knowledge
Unveiling The Mechanics Behind Gpt-3’S Language Generation
Large language models like gpt-3 have garnered a great deal of attention in recent years, with claims that they possess true understanding and knowledge. However, it is important to take a closer look at the reality behind these assertions. In this section, we will explore the mechanics behind gpt-3’s language generation and uncover the distinction between statistical associations and true comprehension.
Gpt-3, which stands for generative pretrained transformer 3, is an advanced language model developed by openai. It is based on deep learning neural networks and utilizes a massive amount of data to generate human-like text. While gpt-3 is undoubtedly an impressive technological achievement, it is crucial to recognize its limitations and understand the underlying processes that drive its language generation capabilities.
Statistical Associations And True Comprehension
At its core, gpt-3 relies on statistical associations to generate coherent and contextually relevant text. It processes vast amounts of text data during its pre-training phase, learning patterns and relationships between words, phrases, and contexts. When provided with a prompt, gpt-3 leverages these learned associations to predict the most probable next word or phrase, resulting in the generation of seemingly coherent and sensible sentences.
However, it is essential to note that gpt-3 lacks genuine understanding or knowledge in the same way humans do. While it can produce impressive outputs, it does not possess the ability to comprehend the meaning or context behind the words it generates.
Instead, gpt-3 merely relies on patterns and statistical correlations to generate text that appears meaningful.
To further illustrate this distinction, let’s explore the key differences between statistical associations and true comprehension:
- Statistical associations:
- Gpt-3 operates based on patterns and statistical correlations.
- It predicts the most probable words or phrases based on its pre-training data.
- The model generates text that seemingly makes sense but lacks genuine understanding.
- True comprehension:
- Humans possess a deep understanding of language and context.
- They can interpret meaning, discern nuances, and apply knowledge to generate coherent text.
- Humans have insight, intuition, and the ability to grasp abstract concepts that gpt-3 lacks.
Understanding this difference allows us to get a more holistic view of gpt-3 and similar large language models. While they excel at generating coherent text, their outputs are fundamentally reliant on statistical associations rather than true comprehension.
Gpt-3’s language generation is a remarkable feat of artificial intelligence, but it is crucial to acknowledge its limitations. While it may produce impressive outputs, it lacks the true understanding and knowledge that humans possess. By unveiling the mechanics behind gpt-3’s language generation, we can achieve a balanced perspective on its capabilities.
Myth 3: Gpt-3 Is Imbued With Unbiased And Ethical Values
Reality vs hype – a balanced look at large language models like gpt-3
Large language models like gpt-3 have garnered significant attention and hype for their ability to generate human-like text. While it’s true that these models have demonstrated remarkable language capabilities, it’s essential to take a closer look at some of the claims surrounding them.
In this blog post section, we will explore the common myths and misconceptions about gpt-3, specifically focusing on the belief that it is imbued with unbiased and ethical values.
Analyzing the impact of bias in training data on gpt-3’s outputs:
- Language models like gpt-3 learn from vast amounts of text data from the internet. However, this training data is not without its flaws and biases. Here’s how bias in training data affects gpt-3’s outputs:
- Biased data: If the training data contains biased language or viewpoints, gpt-3 may unintentionally generate biased responses, perpetuating stereotypes and prejudices.
- Lack of context: Gpt-3 lacks the ability to fully understand and contextualize information it generates. This may lead to the reproduction of biased content without recognizing its harmful implications.
Raising questions about ethical responsibility in ai language models:
- Unconscious biases: Since gpt-3 learns from data created by humans, it inevitably absorbs the biases prevalent in society. This raises ethical questions about the responsibility of developers and researchers to ensure systems like gpt-3 do not amplify or reinforce such biases.
- Unintentional harm: Language models like gpt-3 have the potential to reach a vast audience, making it important to consider the unintended consequences of their outputs. Biased or unethical language generated by gpt-3 could potentially harm individuals or perpetuate misinformation.
While efforts are being made to address some of these concerns, it is crucial to acknowledge the limitations of large language models like gpt-3 and the ongoing need for improvement. Recognizing the potential for bias and ethical ramifications is just one step towards building more responsible and robust ai systems.
As with any powerful technology, it is imperative to approach it with caution, critically evaluate its outputs, and work towards mitigating any unintended negative consequences. By openly discussing the reality of large language models like gpt-3, we can promote a more balanced understanding and responsible development of ai technologies.
The Power And Potential Of Gpt-3 In Various Applications
With the advent of large language models like gpt-3, the field of natural language processing has witnessed a surge in excitement and curiosity. This powerful tool has the potential to revolutionize various industries and applications, enhancing productivity and creativity in ways we could never have imagined.
In this section, we will explore the real-world use cases where gpt-3 shines and how it can be leveraged to address current challenges.
Exploring Real-World Use Cases Where Gpt-3 Shines
Gpt-3 has proven its mettle in a wide range of domains, demonstrating its versatility and ability to generate human-like text in an array of scenarios. Some notable use cases include:
- Customer support: Gpt-3 can assist in providing fast and accurate responses to customer queries, reducing the need for manual intervention. Its ability to understand context and generate coherent replies makes it an ideal tool for improving customer support services.
- Content generation: Gpt-3 can be used to create engaging and informative content across various industries. From blog posts and articles to social media captions, this powerful language model can save time and effort for content creators by generating high-quality text on a given topic.
- Language translation: Gpt-3 can aid in breaking down language barriers by providing accurate translations between different languages. Its proficiency in understanding nuances and context helps in delivering more precise translations, enhancing communication between individuals and businesses globally.
- Virtual assistants: Gpt-3 can be deployed as a virtual assistant, capable of interacting with users through natural language. Its advanced conversational abilities enable it to perform tasks such as scheduling appointments, providing recommendations, and even engaging in creative discussions.
- Programming assistance: Gpt-3 can assist developers in writing code and solving programming problems. By understanding the requirements and context, it can generate code snippets and offer suggestions, significantly improving the efficiency of the coding process.
These are just a few examples of the countless ways gpt-3 can be utilized to streamline processes, augment human capabilities, and drive innovation across various fields.
Leveraging Gpt-3 To Enhance Productivity And Creativity
Gpt-3’s potential to enhance productivity and creativity knows no bounds. Here are some key areas where it can make a significant impact:
- Content curation and summarization: Gpt-3 can assist in curating and summarizing vast amounts of content quickly. By extracting the most relevant information and condensing it into a concise summary, it empowers users to consume information efficiently and make well-informed decisions.
- Creative writing assistance: Gpt-3 can be a writer’s ally, providing inspiration and generating ideas for creative pieces. It can help overcome writer’s block by suggesting plotlines, character arcs, and even dialogue, serving as a valuable tool for authors and content creators.
- Market research and analysis: Gpt-3’s language processing capabilities can aid in conducting market research and analyzing consumer sentiment. By analyzing large datasets and identifying trends, businesses can gain valuable insights to drive decision-making and improve their marketing strategies.
- Personalized recommendations: Gpt-3 can leverage data from user interactions and preferences to provide personalized recommendations. From suggesting movies and books to recommending products and services, it enhances user experiences by delivering tailored suggestions based on individual preferences.
By harnessing the power of gpt-3, individuals and organizations can unlock new levels of productivity and creativity, transforming the way we work and engage with technology.
Gpt-3 offers immense potential in various applications, revolutionizing industries and empowering individuals to achieve more. As the capabilities of large language models continue to evolve, we can expect to witness even more exciting use cases and groundbreaking innovations in the near future.
The possibilities are endless, and the journey has just begun.
Addressing Ethical Implications And Concerns
Reality vs hype – a balanced look at large language models like gpt-3
Large language models like gpt-3 have gained significant attention in recent years due to their ability to generate human-like text. These models have been used for a wide range of applications, from assisting in content creation to powering virtual assistants.
However, with their remarkable capabilities come ethical implications and concerns that need to be addressed. In this section, we will explore how gpt-3’s training ensures fairness and transparency, and discuss the steps taken to mitigate bias and unethical use cases.
Ensuring Fairness And Transparency In Gpt-3’S Training
Despite the impressive capabilities of gpt-3, the training process must prioritize fairness and transparency. Here are key points to consider:
- Algorithmic accountability: Gpt-3’s development team recognizes the importance of transparency in ai algorithms. Efforts are made to document the training methods and data sources used for training the model, allowing researchers to understand its limitations and potential biases.
- Openai’s engagement with the research community: Openai actively encourages external researchers to audit their models and provide feedback. This collaborative approach helps identify and rectify any bias or unfairness that might arise during the training process.
- Public discussions on model behavior: Openai believes in including public input in decisions regarding system behavior and deployment policies. This approach ensures diverse perspectives are considered, and helps avoid concentration of power and undue influence.
- Documentation and guidelines for usage: Openai provides clear guidelines and documentation for those who use gpt-3, ensuring that users are well-informed about potential ethical concerns and the responsible use of the system.
Discussing Steps Taken To Address Bias And Mitigate Unethical Use Cases
To address bias and mitigate the potential misuse of gpt-3, openai has implemented several measures. Here are the key points to consider:
- Continuous research and improvement: Openai is committed to ongoing research and development to address biases in gpt-3 and reduce its susceptibility to unethical use. Improvements to the training methods and fine-tuning processes are being made to minimize biases in the model’s outputs.
- User feedback and human reviewers: Openai actively seeks feedback from users to understand and rectify any unwanted or biased behavior exhibited by gpt-3. The use of human reviewers also helps in evaluating the model’s outputs and ensuring its alignment with ethical guidelines.
- Reducing harmful outputs: Openai is working towards reducing the generation of harmful or objectionable content by refining the model’s behavior and providing clearer instructions to human reviewers during the training process.
- Ethical deployment policies: Openai is committed to deploying gpt-3 in a manner that minimizes risks and maximizes societal benefit. They are actively exploring partnerships to conduct third-party audits and ensure that the technology is used for positive outcomes without causing harm.
Addressing the ethical implications and concerns associated with large language models like gpt-3 is crucial to building trust in the technology. Openai’s emphasis on fairness, transparency, continuous improvement, and responsible deployment paves the way for a more ethical and inclusive future in ai.
Future Possibilities And Limitations Of Gpt-3
Reality vs hype – a balanced look at large language models like gpt-3
Large language models like gpt-3 have generated a lot of excitement and anticipation in recent years. These models possess immense potential when it comes to transforming various industries and revolutionizing the way we interact with technology. However, it is essential to take a balanced approach and evaluate the future possibilities and limitations of gpt-3.
Discovering The Ongoing Research And Development To Improve Gpt-3
While gpt-3 has already demonstrated impressive capabilities, ongoing research and development efforts are focused on further enhancing its performance and addressing its limitations. Key points to consider include:
- Training data refinement: Improving the quality and diversity of training data can significantly enhance the model’s understanding of complex concepts and cultural nuances. This ongoing effort aims to refine gpt-3’s ability to generate more accurate and contextually appropriate responses.
- Fine-tuning and customization: By allowing users to fine-tune gpt-3 for specific domains or tasks, researchers are exploring ways to make the model more adaptable and tailored to individual needs. This approach can lead to enhanced performance and better integration into specific industries or professional settings.
- Reducing computational requirements: Gpt-3’s training and inference processes currently require significant computational resources. Researchers are actively exploring strategies to improve efficiency and reduce the computational requirements, making large language models like gpt-3 more accessible and practical for a wider range of applications.
- Ethical considerations and bias: Addressing ethical concerns and minimizing bias in the outputs of large language models is an ongoing area of research. Efforts are being made to ensure that the generated content is fair, unbiased, and adheres to ethical guidelines, avoiding potential pitfalls related to misinformation or harmful content generation.
Recognizing The Boundaries And Challenges That Lie Ahead
While gpt-3 holds immense promise, it is vital to acknowledge the limitations and challenges that lay ahead on its path to further development. Consider the following points:
- Contextual understanding and common sense: Gpt-3 may struggle to grasp the wider context of a conversation or lack basic common sense reasoning. It is important to remember that gpt-3 is a machine learning model that lacks true comprehension of language and relies on patterns from its training data.
- Reliance on training data: The model’s performance is highly dependent on the quality and diversity of its training data. As gpt-3 is trained on existing datasets, it may reflect and perpetuate biases present in those datasets. This can lead to biased or inaccurate outputs that need to be carefully addressed.
- Interpretability and explainability: Gpt-3’s decision-making process is often considered a black box, making it challenging to understand why certain responses are generated. Achieving improved interpretability and explainability is a crucial aspect for the widespread adoption and acceptance of large language models.
- Real-world limitations: Although the capabilities of gpt-3 are impressive, it is crucial to recognize that there are real-world limitations that may hinder its adoption in certain applications. Factors such as cost, computational requirements, and processing time can limit the practicality of using gpt-3 in certain scenarios.
By exploring ongoing research and development efforts, as well as recognizing the limitations and challenges, we can gain a clearer understanding of the future possibilities and boundaries of large language models such as gpt-3. This balanced view is essential to harnessing the potential of these models while navigating through any associated caveats responsibly.
Embracing The Potential Of Gpt-3 While Acknowledging Its Limitations
Large language models like gpt-3 have garnered significant attention in recent years, offering a glimpse into the exciting possibilities of artificial intelligence (ai). Gpt-3, developed by openai, demonstrates immense potential when it comes to generating human-like text, answering questions, and even performing creative tasks.
However, it is crucial to approach these models with a balanced perspective, recognizing both their strengths and limitations. In this section, we will explore the considerations for using gpt-3 in various industries and fields, as well as discuss the importance of setting realistic expectations for ai language models like gpt-3.
Considerations For Using Gpt-3 In Various Industries And Fields
Gpt-3’s versatility makes it applicable to a wide range of industries and fields. However, before embracing the potential of gpt-3, it is crucial to consider the following factors:
- Data quality and bias: Gpt-3 relies heavily on the data it is trained on, sometimes leading to biased or inaccurate outputs. Therefore, ensuring the quality and diversity of the training data is essential to obtain reliable results.
- Domain-specific expertise: Gpt-3 may struggle when it comes to highly specialized or technical domains. It is important to assess whether the language model has sufficient training in the specific field you are working in to provide accurate and relevant information.
- Ethical implications: As with any ai technology, using gpt-3 raises ethical considerations. The potential for misinformation, the need to ensure data privacy, and the accountability for the generated content should all be taken into account.
- Cost and infrastructure: Implementing gpt-3 may require significant computational resources and financial investment. It is essential to assess whether the potential benefits outweigh the associated costs.
Setting Realistic Expectations For Ai Language Models Like Gpt-3
While gpt-3 is undeniably impressive, it is crucial to set realistic expectations for what it can achieve. Here are some important points to keep in mind:
- Imperfect outputs: Gpt-3 is not infallible, and it can sometimes produce inaccurate or nonsensical content. Understand that it is a tool that augments human capabilities rather than replaces them entirely.
- Lack of contextual understanding: Gpt-3 lacks true understanding of context and may produce unreliable responses when faced with ambiguous queries or complex situations. It is important to carefully review and verify its outputs.
- Guarding against bias: Since gpt-3 learns from existing data, it can inadvertently adopt biases present in the training data. Vigilance is necessary to ensure that the generated content is fair, unbiased, and suitable for diverse audiences.
- Supervised fine-tuning: Gpt-3’s performance can be enhanced through supervised fine-tuning, where experts review and correct its outputs. This process helps improve the model’s accuracy and reliability.
Gpt-3 presents immense possibilities for various industries and fields, but it is crucial to approach it with realistic expectations and understanding of its limitations. By considering the specific needs of your industry, setting realistic goals, and using gpt-3 as a tool rather than a perfect solution, you can harness its potential effectively.
Frequently Asked Questions Of Reality Vs Hype – A Balanced Look At Large Language Models Like Gpt-3
What Is Gpt-3 And How Does It Work?
Gpt-3, which stands for generative pre-trained transformer 3, is a large language model that uses deep learning to generate human-like text based on given prompts.
How Does Gpt-3 Differ From Previous Language Models?
Gpt-3 is much larger and more powerful than previous language models, with 175 billion parameters. This allows it to generate more coherent and contextually accurate text.
What Are The Potential Applications Of Gpt-3?
Gpt-3 has a wide range of potential applications, including chatbots, content generation, language translation, code writing, and virtual assistants.
Can Gpt-3 Replace Human Creativity And Intelligence?
While gpt-3 is impressive in its ability to generate text, it cannot replace human creativity and intelligence. It is a tool that can assist humans in various tasks, but it does not possess human-like understanding or consciousness.
What Are The Limitations And Challenges Of Using Gpt-3?
Gpt-3 has limitations in terms of biases in training data, potential ethical concerns, and the need for large amounts of computational resources. It also struggles with understanding context and can sometimes produce inaccurate or nonsensical outputs.
Conclusion
To sum up, it’s clear that large language models like gpt-3 have generated a lot of excitement and hype in recent times. They showcase immense potential for a wide range of applications, from customer service to content creation. However, it’s important to approach these models with a balanced perspective.
While they have demonstrated impressive capabilities, they also have their limitations and ethical considerations. It’s crucial to remember that language models are not infallible and can produce biased or inaccurate outcomes. They require continuous monitoring and fine-tuning to ensure their outputs align with desired goals.
Additionally, the access and affordability of these models can be a concern, especially for smaller businesses or individuals. That being said, the future is brighter than ever for large language models, as they pave the way for innovative advancements in ai technology.
As researchers continue to refine and enhance these models, we can expect more sophisticated and reliable results. By keeping a critical eye and considering the ethical implications, we can harness the true potential of large language models and make them a valuable asset in our digital landscape.