Trust in ai is crucial for fair, safe, and reliable systems, which can be achieved through adherence to principles and best practices. Artificial intelligence (ai) has become an integral part of our daily lives, from virtual assistants like siri and alexa to autonomous vehicles.
However, as ai continues to advance, so does the need for trust in its capabilities. Trust in ai systems is essential for ensuring fairness, safety, and reliability. To achieve this trust, developers and organizations must adhere to principles and practices that promote transparency, accountability, and ethical decision-making.
This includes clear communication about how ai systems work, addressing biases and discriminatory outcomes, and implementing robust testing and validation procedures. By following these principles and practices, we can build ai systems that are trusted, ensuring their responsible and beneficial use in various domains.
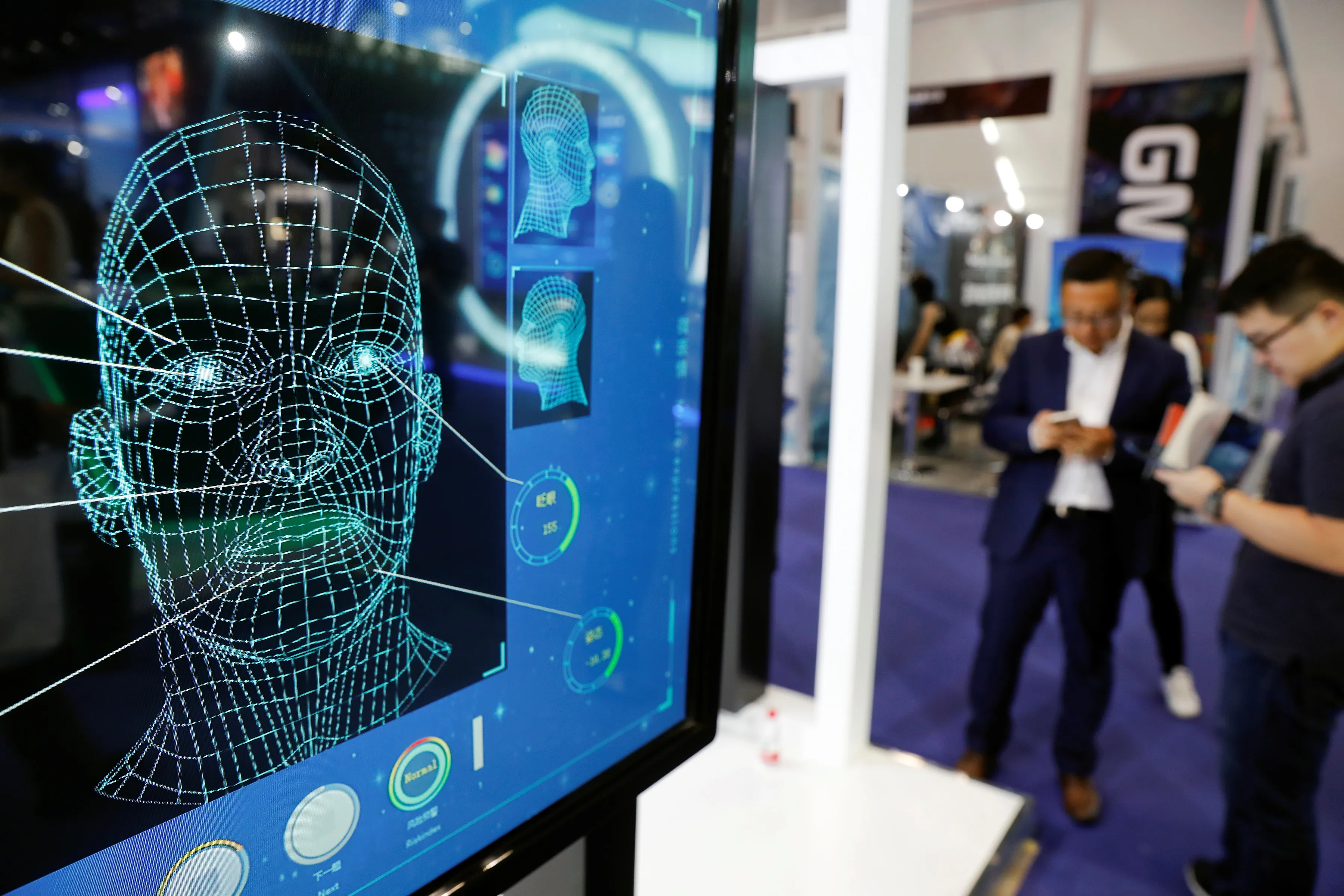
Credit: www.brookings.edu
The Importance Of Trust In Ai
Trust As A Crucial Factor In Ai Adoption
As artificial intelligence (ai) continues to advance and permeate various industries, trust becomes an indispensable factor for its widespread adoption. Trust in ai systems is crucial for organizations and individuals to feel confident in relying on these technologies. Without trust, the potential benefits of ai may be hindered.
In this section, we will explore the challenges of building trust in ai systems and the benefits that come from incorporating trustworthy ai practices.
The Challenges Of Building Trust In Ai Systems:
Building trust in ai systems is not without its challenges. Here are some key points to consider:
- Transparency: Lack of transparency in ai algorithms can lead to skepticism and uncertainty. Users may be hesitant to trust systems whose inner workings are not fully understood.
- Bias and fairness: Ai systems are trained using data, and if that data contains biases, it can lead to biased outcomes. Ensuring fairness and mitigating bias is essential to building trust in ai systems.
- Explainability: Ai models often produce results without providing clear explanations. This lack of explainability can make it difficult for users to trust and understand the decisions made by ai systems.
- Security and privacy: The potential for misuse or breaches of ai systems is a significant concern for users. Ensuring robust security measures and safeguarding privacy are crucial elements in building trust.
The Benefits Of Trustworthy Ai Systems:
Trustworthy ai systems offer several benefits that contribute to their adoption and impact. Consider the following points:
- Enhanced performance: Trustworthy ai systems can consistently deliver reliable and accurate results, improving their overall performance.
- Ethical decision-making: By addressing bias and ensuring fairness, trustworthy ai systems have the potential to make more ethical decisions, promoting fairness and inclusivity.
- Increased user adoption: When users trust ai systems, they are more likely to embrace them and incorporate them into their workflows, leading to increased adoption rates and overall utilization.
- Reduced legal and reputational risks: Trustworthy ai systems can help organizations mitigate legal and reputational risks associated with biased or unethical outcomes, safeguarding their integrity.
By acknowledging the challenges of building trust in ai systems and understanding the benefits of trustworthy practices, organizations can prioritize the development and implementation of ai systems that inspire confidence and enable responsible and reliable use of these technologies. Trust in ai is not just a desirable aspect; it is a fundamental prerequisite for its successful integration into various domains.
Ethical Principles For Ensuring Trust In Ai
Trust in ai – principles and practices for fair, safe and reliable systems
Transparency And Explainability In Ai Algorithms
Transparency and explainability are crucial factors in building trust in ai systems. Here are key points to consider:
- Understanding ai decisions: Users should be able to understand why ai systems make specific decisions or recommendations.
- Clear explanations: Ai algorithms should provide clear and concise explanations for their outputs, ensuring transparency in the decision-making process.
- Traceability: It’s important to have a mechanism in place to trace and understand how the ai algorithms arrived at their conclusions.
- Openness: Encourage companies and organizations to be transparent about the ai algorithms they use and provide clear documentation about their functionality and limitations.
Fairness And Accountability In Decision-Making
Fairness and accountability are crucial ethical principles for ensuring trust in ai systems. Here are the key points to consider:
- Avoiding bias: Ai algorithms should be designed in a way that avoids bias or discrimination against any specific group of individuals.
- Data representativeness: Ensure the training data used for ai models is diverse and representative of the population, to avoid biased outcomes.
- Regular auditing: Regularly audit ai systems to identify and correct any potential biases or unfairness in decision-making.
- Accountability measures: Establish clear accountability measures, making sure that the responsible parties are identifiable and can be held accountable for any negative consequences of ai systems.
Privacy And Data Protection In Ai Systems
Privacy and data protection are essential aspects of trustworthy ai systems. Here are key points to consider:
- Data anonymization: Ensure that personal data used in ai systems is anonymized and cannot be easily linked back to individuals.
- Informed consent: Obtain proper informed consent from users before collecting and processing their data for ai purposes.
- Secure storage: Safeguard user data through secure storage and encryption techniques to protect it from unauthorized access or breaches.
- Data minimization: Collect and use only the necessary data for ai systems, minimizing the risk of privacy infringements.
Robustness And Reliability In Ai Models
Robustness and reliability are fundamental for building trust in ai models. Here are key points to consider:
- Thorough testing: Ai models should undergo comprehensive testing to ensure their robustness and reliability in different scenarios.
- Adaptation to changing environments: Ai systems should be designed to adapt and perform well in dynamically changing environments.
- Risk mitigation: Develop mechanisms to mitigate potential risks and failures of ai systems, ensuring that they are reliable and safe to use.
- Continuous monitoring: Regularly monitor ai models’ performance and reliability to detect and address any issues or deviations.
By adhering to these ethical principles, we can foster trust in ai systems, ultimately creating fair, safe, and reliable solutions that positively impact various domains.
Best Practices For Trustworthy Ai Systems
Trust in ai systems is crucial for their widespread adoption and acceptance. To ensure the trustworthiness of ai, certain best practices need to be followed. This section focuses on the key principles and practices that can help in building and maintaining trustworthy ai systems.
Increased Interpretability Of Ai Models
- Ai models can often be complex and difficult to understand. Increased interpretability of these models helps in enhancing transparency and trust.
- Explanation techniques such as feature importance analysis, rule extraction, and visualizations can provide insights into how ai models reach their decisions.
- Clear and intelligible explanations can lead to better understanding, identification of biases, and mitigation of potential risks.
- Documentation of ai models’ decisions and justifications can aid in their review and enable stakeholders to assess and verify the system’s behavior.
Diverse And Inclusive Datasets
- Ai systems should be trained on diverse and inclusive datasets, representing a wide range of individuals, experiences, and perspectives.
- Diverse datasets help in reducing biases and ensuring fairness in ai systems. It enables the development of more inclusive and representative models.
- Data collection efforts should strive to address underrepresented groups and avoid reinforcing existing biases or stereotypes.
- Regular evaluation and testing of datasets for potential biases, both explicit and implicit, should be undertaken to ensure the fairness of ai systems.
Regular Monitoring And Auditing Of Ai Systems
- Ongoing monitoring and auditing of ai systems are essential to ensure their continued trustworthiness.
- Regular evaluation of the system’s performance, behavior, and impact is necessary to identify and mitigate potential risks and biases.
- Continual assessment of data quality, integrity, and sources is important to maintain the credibility of ai systems.
- Feedback loops, user feedback, and continuous learning should be incorporated into ai systems to improve their reliability and responsiveness.
Collaborative Efforts And Partnerships For Responsible Ai Development
- Responsible ai development requires collaborative efforts and partnerships among various stakeholders, including researchers, developers, policymakers, and end-users.
- Collaboration helps in sharing knowledge, insights, and best practices for building and deploying trustworthy ai systems.
- Public-private partnerships can facilitate the development of standards, guidelines, and regulations for ai governance and ethics.
- Engaging diverse perspectives and including input from affected communities can lead to better decision-making and more inclusive ai systems.
Ensuring trust in ai systems requires the adoption of best practices such as increased interpretability of ai models, using diverse and inclusive datasets, regular monitoring and auditing, and collaborative efforts among stakeholders. Following these principles and practices will contribute to the development of fair, safe, and reliable ai systems, fostering trust among users and society as a whole.
Regulatory Frameworks For Ensuring Trust In Ai
Trust in ai – principles and practices for fair, safe and reliable systems
Technological advancements have led to the widespread adoption of artificial intelligence (ai) in various industries. As ai becomes more integrated into our daily lives, ensuring trust in its deployment becomes paramount. This requires the establishment of regulatory frameworks that promote fair, safe, and reliable ai systems.
In this section, we will explore the different aspects of regulatory frameworks that contribute to trust in ai.
International Standards And Guidelines For Ai Ethics
International bodies and organizations have recognized the need for ethical guidelines to govern ai development and deployment. These standards and guidelines provide a framework for organizations and governments to follow, ensuring responsible and accountable ai practices. Key points include:
- The development of guidelines addressing transparency, accountability, and fairness in ai systems.
- The establishment of principles that promote non-discrimination, privacy protection, and data security.
- Encouraging interdisciplinary collaboration to develop comprehensive frameworks that consider legal, social, and ethical implications.
Government Regulations And Policies For Ai Governance
Governments play a crucial role in shaping the ethical and trustworthy use of ai technologies. To ensure public trust, governments have started implementing regulations and policies that govern ai development and deployment. Some key aspects include:
- The creation of regulatory bodies or committees to oversee ai governance and address societal concerns.
- Compliance requirements for organizations working with ai technologies.
- Regulations pertaining to data protection, user consent, and algorithmic transparency.
- Guidelines for ai use in critical sectors such as healthcare, finance, and transportation.
Industry Initiatives And Self-Regulation In Ai Development
Beyond government regulations, the ai industry itself has taken steps towards self-regulation to ensure responsible ai development. Industry initiatives and collaborations contribute to building trust in ai systems. Important considerations include:
- The establishment of ethical guidelines and codes of conduct specific to ai developers, data scientists, and engineers.
- The formation of consortiums and alliances that encourage knowledge sharing and best practices.
- Practices such as independent audits and certifications to assess the ethical implications of ai systems and algorithms.
Public Awareness And Engagement In Ai Decision-Making
Promoting public awareness and engagement in ai decision-making processes is essential to establish trust in ai systems. Informed public participation ensures that ai deployment aligns with societal values and addresses potential biases or unfairness. Key points involve:
- Encouraging public dialogue and education regarding ai technologies, their benefits, and risks.
- Incorporating diverse perspectives in the development and evaluation of ai systems.
- Ensuring transparency and clarity in communicating ai decision-making processes to the public.
Regulatory frameworks play a vital role in establishing trust in ai systems. International standards and guidelines, government regulations, industry self-regulation, and public engagement are all integral to building fair, safe, and reliable ai technologies. By adhering to these principles and practices, we can foster an environment where ai is developed and used in a responsible and trustworthy manner.
Frequently Asked Questions On Trust In Ai – Principles And Practices For Fair, Safe And Reliable Systems
Q: What Are The Key Principles Of Trustworthy Ai Systems?
A: trustworthy ai systems are built upon principles of fairness, safety, reliability, transparency, and accountability.
Q: How Can Ai Systems Ensure Fairness?
A: ai systems can ensure fairness by utilizing unbiased algorithms, avoiding biased data, and promoting diversity in the development process.
Q: What Safety Measures Are Implemented In Ai Systems?
A: ai systems prioritize safety by conducting rigorous testing, implementing fail-safe mechanisms, and adhering to ethical guidelines.
Q: How Can Ai Systems Be Reliable?
A: ai systems can be made reliable through robust training, continuous monitoring, and regular updates to adapt to changing conditions.
Q: Why Is Transparency Important In Ai Systems?
A: transparency in ai systems enables users to understand the decision-making process, build trust, and detect potential biases or errors.
Conclusion
Trust in ai is essential for the widespread adoption and utilization of artificial intelligence systems. As we have explored in this blog post, principles and practices for fair, safe, and reliable ai systems are crucial. By adhering to these principles, we can ensure that ai technologies are developed and deployed in a responsible and ethical manner.
Transparency, accountability, and inclusivity must be at the forefront of ai development and implementation. It is important for organizations and policymakers to establish guidelines and regulations that promote trust and address concerns related to bias, security, and privacy. Collaboration between industry, academia, and government is vital for further advancement and evolution of trustworthy ai.
By prioritizing fairness, safety, and reliability, we can build a future where ai enhances our lives while upholding our values and ethics.