A knowledge graph is a powerful tool that helps organize and display information in a visual and interactive way. It enhances search engine results by providing relevant and accurate information directly in the search results page.
With a knowledge graph, users can quickly find answers to their questions without having to click through multiple websites. In addition, knowledge graphs are essential for voice search and artificial intelligence applications, as they provide structured and easily accessible data.
Let’s delve deeper into the world of knowledge graphs and explore their benefits, working mechanism, and how they contribute to improving search experiences for users.
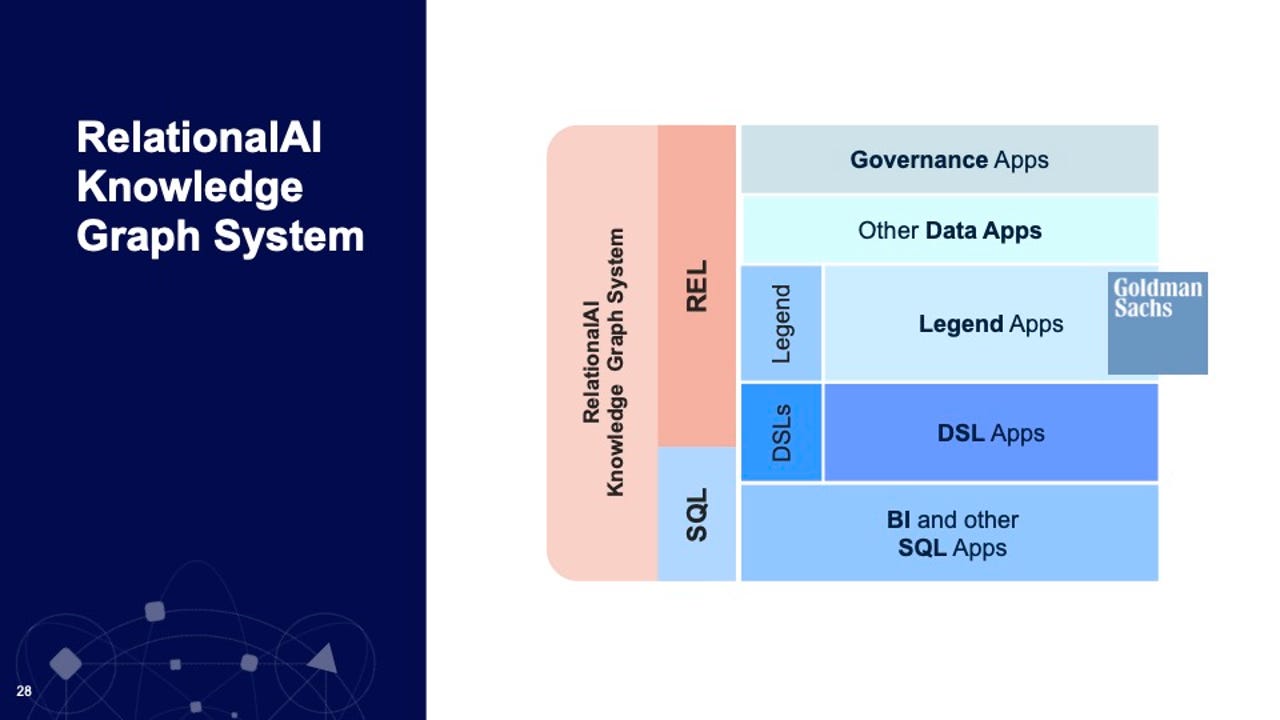
Credit: www.zdnet.com
What Are Knowledge Graphs?
Definition And Overview Of Knowledge Graphs
In the digital landscape, knowledge graphs have emerged as a powerful tool for organizing and representing information in a structured manner. Essentially, a knowledge graph is a massive knowledge base that stores and connects a wide range of data points, creating a web of relationships between them.
This interconnectedness enables the graph to uncover meaningful insights and provide more relevant information to users. Let’s delve deeper into how knowledge graphs store and represent information.
How Knowledge Graphs Store And Represent Information
Knowledge graphs use a graph structure comprising nodes (entities) connected by edges (relationships). These nodes represent specific entities, such as people, places, events, or concepts. The edges, on the other hand, illustrate the connections and associations between these entities. Here are some key points to understand about how knowledge graphs store and represent information:
- Entity relationships: Knowledge graphs capture the relationships between entities by mapping connections between them. For example, a knowledge graph might connect an artist node with album nodes to signify that the artist has released multiple albums.
- Ontology: Knowledge graphs often incorporate an ontology, which is a formal representation of the categories, properties, and relationships within a specific domain. The ontology adds depth and structure to the knowledge graph, making it more navigable and comprehensive.
- Contextual information: Knowledge graphs also incorporate contextual information, such as attributes, metadata, and descriptions, alongside the entities and relationships. This contextual information enhances the understanding of the data and provides additional insights to both users and machines.
Examples Of Popular Knowledge Graphs
One exemplary knowledge graph that prominently stands out is the google knowledge graph. With its vast array of interconnected information, the google knowledge graph plays a significant role in enhancing search results and answering search queries effectively. Here are some other notable examples of popular knowledge graphs:
- Wikidata: As a collaborative knowledge graph, wikidata serves as a central hub for linked data and structured information from various wikimedia projects. Its extensive dataset powers information boxes across wikipedia articles.
- Facebook open graph: The facebook open graph is a powerful tool for extracting and organizing data from users’ interactions on the platform. It allows users to easily connect and share information, hobbies, and interests with others.
- Microsoft academic graph: Focused on scholarly research, the microsoft academic graph brings together publications, authors, institutions, and other research-related entities into one interconnected graph. It enriches the academic search experience and facilitates comprehensive analysis.
Knowledge graphs offer an organized and interconnected approach to storing and representing information. Their ability to capture entity relationships, incorporate ontologies, and provide contextual information makes them invaluable in various domains. With examples such as the google knowledge graph, the potential for knowledge graphs to enhance information retrieval and knowledge discovery is profound.
Why Use Knowledge Graphs?
The Benefits Of Using Knowledge Graphs In Various Industries
Knowledge graphs have emerged as a powerful tool in the world of data management and analysis. With their ability to organize and synthesize complex information, it’s no wonder that knowledge graphs are being used across various industries. Let’s explore the key benefits of using knowledge graphs in different sectors:
Improved Data Integration And Understanding
- Knowledge graphs enhance data integration by connecting diverse data sources and creating a unified view. This allows businesses to analyze information that was previously siloed.
- By organizing data in a semantic structure, knowledge graphs enable better data understanding. Relationships between entities become clear, leading to insights that might have been overlooked otherwise.
- With knowledge graphs, data can be efficiently queried, reducing the time spent on data preparation and increasing productivity.
Case Studies Showcasing Successful Implementations Of Knowledge Graphs
- Healthcare: The healthcare industry has embraced knowledge graphs to improve patient care and research outcomes. By integrating patient data, medical records, and research findings, knowledge graphs enable accurate diagnosis, personalized treatment plans, and better epidemiological insights.
- E-commerce: Knowledge graphs have revolutionized the e-commerce sector by delivering personalized product recommendations and enhancing customer experiences. By analyzing customers’ preferences, browsing histories, and previous purchases, knowledge graphs help businesses tailor their offerings and increase sales.
- Digital assistants: Virtual assistants like siri and alexa rely on knowledge graphs to understand and respond to user queries effectively. By leveraging structured knowledge, these assistants can provide accurate and contextually relevant information to users.
- Recommendation systems: Streaming platforms and content providers use knowledge graphs to deliver personalized recommendations to their users. By analyzing user preferences, viewing history, and content metadata, knowledge graphs help platforms suggest relevant content, leading to increased user engagement.
Knowledge graphs offer numerous benefits across various industries. From improved data integration and understanding to successful real-world implementations, these powerful tools facilitate data analysis, enhance decision-making, and drive innovation. As businesses continue to realize the potential of knowledge graphs, we can expect further advancements and novel use cases in the future.
How Do Knowledge Graphs Work?
Knowledge graphs are increasingly becoming a fundamental component of modern technology, playing a crucial role in powering search engines, voice assistants, and recommendation systems. But how do knowledge graphs actually work? In this section, we will explore the key components and structure of a knowledge graph, the role of ontologies, schema, and linked data, as well as the significance of graph databases in knowledge graph technology.
The Key Components And Structure Of A Knowledge Graph
A knowledge graph is a powerful representation of information that connects different entities and their relationships in a structured manner. Here are the key points to understand about the structure and components of a knowledge graph:
- Entities: Knowledge graphs are built upon entities, which can be anything from people and places to concepts and events. Each entity is represented by a node in the graph.
- Relationships: The relationships between entities are the backbone of a knowledge graph. They define the connections and associations between different nodes, enabling the graph to capture the intricate web of knowledge.
- Attributes: Entities in a knowledge graph are enriched with attributes or properties that provide additional details about them. These attributes could include things like names, descriptions, dates, or any other relevant information.
- Hierarchical structure: Knowledge graphs often possess a hierarchical structure, with broader concepts at the top and more specific ones below. This allows for better organization and categorization of information within the graph.
- Inference and reasoning: Knowledge graphs often incorporate inference and reasoning capabilities, enabling them to generate new insights by drawing logical conclusions from the existing data.
The Role Of Ontologies, Schema, And Linked Data In Knowledge Graphs
To enhance the organization and interpretation of data within a knowledge graph, several additional elements come into play. These include ontologies, schema, and linked data. Here’s a breakdown of their respective roles:
- Ontologies: Ontologies provide a formal and explicit specification of the concepts, relationships, and properties within a specific domain. They act as a foundational framework for knowledge graphs, ensuring consistency and enabling better understanding and interoperability of data.
- Schema: A schema defines the structure and organization of a knowledge graph, specifying the types of entities, relationships, and attributes that can be included. It serves as a blueprint for the graph, facilitating data integration and ensuring data quality.
- Linked data: Linked data is a method of publishing structured data on the web, making it interlinked and machine-readable. It allows knowledge graphs to seamlessly connect to external data sources, expanding their scope and enriching their information.
Explanation Of Graph Databases And Their Significance In Knowledge Graph Technology
Graph databases play a critical role in knowledge graph technology, offering robust storage and querying capabilities specifically designed for interconnected data. Here’s why they are significant:
- Efficient relationships: Graph databases excel at managing and navigating complex relationships between entities, providing fast and efficient traversal of the graph. This allows for quick retrieval of indirect connections, enabling more sophisticated knowledge graph applications.
- Flexible data model: The flexible data model of graph databases accommodates the evolving nature of knowledge graphs. As new entities and relationships emerge, the graph can easily be extended, making it highly adaptable to changing data requirements.
- Query performance: With graph-specific indexing and query optimization techniques, graph databases deliver exceptional query performance, even when dealing with vast amounts of interconnected data. This ensures that knowledge graphs can be queried effectively, providing timely and accurate results.
- Scalability: Graph databases are designed to scale horizontally, allowing knowledge graphs to grow in size while maintaining high performance. This scalability is crucial for handling larger and more complex real-world datasets.
Knowledge graphs are structured representations of information, powered by entities, relationships, attributes, and inference capabilities. They benefit from ontologies, schema, and linked data for better organization and interoperability. Graph databases provide the necessary storage and querying infrastructure, enabling efficient traversal, flexibility, performance, and scalability.
With these components working together, knowledge graphs become powerful tools for organizing and harnessing complex knowledge. So, now that you understand how knowledge graphs work, let’s delve deeper into their benefits and applications!
Building And Implementing Knowledge Graphs
Knowledge graphs are powerful tools that help organize and connect information in a structured manner, enabling businesses to extract valuable insights and improve decision-making processes. If you’re considering building and implementing a knowledge graph for your organization, it’s important to understand the steps involved, as well as the tools and best practices to ensure success.
In this section, we’ll explore the key aspects of building and implementing knowledge graphs.
The Steps Involved In Building A Knowledge Graph:
Building a knowledge graph involves several key steps, which include:
- Identify the domain: Start by defining the domain or subject area for your knowledge graph. This could be a specific industry, a company’s internal knowledge, or even a broader domain like healthcare or finance.
- Gather data: Collect relevant data from various sources such as databases, spreadsheets, apis, and documents. Ensure that the data you gather is accurate, comprehensive, and represents the information you want to include in your knowledge graph.
- Clean and preprocess the data: Before integrating the data into your knowledge graph, it’s crucial to clean and preprocess it. This involves removing duplicates, correcting errors, standardizing formats, and transforming the data into a consistent structure.
- Modeling and ontology design: Define the entities, relationships, and properties that will form the foundation of your knowledge graph. This step includes creating an ontology, which represents the concepts and their relationships within your domain.
- Data integration: Integrate the preprocessed data into your knowledge graph by mapping the entities, attributes, and relationships to their corresponding ontology elements. This step ensures that the data is structured and organized in a meaningful way.
- Knowledge graph construction: Build the actual knowledge graph by connecting the entities and relationships based on the defined ontology and integrated data. This step involves linking entities, assigning properties, and establishing relationships between nodes.
- Querying and visualization: Once your knowledge graph is constructed, you can query and visualize the data to extract insights and gain a better understanding of the information it contains. Utilize query languages like sparql or graph visualization tools to interact with and present the data.
Tools And Technologies For Creating And Managing Knowledge Graphs:
Several tools and technologies can aid in creating and managing knowledge graphs. Some notable ones include:
- Apache jena: An open-source java framework for building semantic web and linked data applications. Jena provides apis for rdf and sparql, making it suitable for knowledge graph construction.
- Neo4j: A graph database management system that allows for efficient storage, querying, and analysis of knowledge graphs. Neo4j’s graph algorithms and query language (cypher) facilitate complex graph traversal and exploration.
- Google knowledge graph api: Google provides an api that allows developers to access its vast repository of structured data. Leveraging this api can enhance your knowledge graph by incorporating external knowledge and expanding its breadth.
- Openrefine: A powerful tool for data cleaning and transformation. Openrefine facilitates the preprocessing and standardization of data, making it easier to integrate into a knowledge graph.
Best Practices For Implementing Knowledge Graphs In Different Domains:
Implementing knowledge graphs successfully requires careful consideration of the domain-specific requirements. Here are some best practices to bear in mind:
- Define clear business goals: Identify the specific objectives and use cases you want to address with your knowledge graph. This will guide the design and development process, ensuring that it aligns with your organization’s needs.
- Involve domain experts: Collaborate with domain experts who possess in-depth knowledge of the subject area. Their expertise will help in defining accurate ontologies, relationships, and important data points.
- Continuously update and expand: Knowledge graphs should be dynamic and evolve over time. Regularly update and expand your knowledge graph to incorporate new data, as well as changes in the domain or business requirements.
- Leverage linked open data: Explore existing linked open data sources that align with your domain, such as dbpedia or wikidata. Integrating these external data sources can enrich your knowledge graph and provide additional context.
- Ensure data quality and governance: Establish data quality standards and governance practices to maintain the integrity and accuracy of your knowledge graph. Regularly validate and monitor the data to avoid errors or inconsistencies.
By following these steps, utilizing appropriate tools and technologies, and adhering to best practices, you can build and implement a robust knowledge graph tailored to your organization’s unique needs. A well-constructed knowledge graph has the potential to unlock valuable insights and drive innovation across various domains.
Frequently Asked Questions Of Everything You Need To Know About Knowledge Graphs
What Is A Knowledge Graph?
A knowledge graph is a database that stores structured information about entities, their relationships, and attributes.
How Does A Knowledge Graph Work?
A knowledge graph retrieves information by connecting the relationships between entities, enabling semantic search and providing contextual information.
Why Is A Knowledge Graph Important For Seo?
A knowledge graph helps search engines understand queries better, improve search results accuracy, and enhance the overall user experience.
Can I Optimize My Website For Knowledge Graphs?
Yes, you can optimize your website by using structured data markup, schema. org, and providing clear and relevant information to improve your chances of appearing in knowledge graphs.
How Can Knowledge Graphs Benefit Businesses?
Knowledge graphs can enhance brand visibility, improve search rankings, drive organic traffic, and provide relevant information to potential customers.
Conclusion
Knowledge graphs have revolutionized the way we interact with information on the internet. They enhance search engine results by organizing data and making connections between different entities. By understanding the context of a search query, knowledge graphs provide users with more relevant and concise answers, saving time and effort.
Whether you’re a business looking to improve your online presence or a curious individual seeking reliable information, knowledge graphs are an important tool to understand. They enable a better understanding of complex concepts and help users navigate through vast amounts of information effortlessly.
As search engines continue to evolve, incorporating more knowledge graphs into their algorithms, it is crucial to stay updated and optimize your content to rank higher. Embrace the power of knowledge graphs and harness its potential to improve user experience, boost visibility, and drive organic traffic to your website.
Start exploring their remarkable benefits today.