Imitation learning in ai allows agents to mimic behavior for accurate decision-making and problem-solving. We will explore the concept of imitation learning and its significance in artificial intelligence.
Imitation learning enables ai agents to learn from demonstrations and imitate the actions of expert humans or other agents. By observing and replicating successful behavior, agents can acquire new skills and improve their performance in various tasks. We will delve into the different approaches and techniques used in imitation learning, including behavioral cloning, inverse reinforcement learning, and generative adversarial imitation learning.
Through a deeper understanding of imitation learning, we can appreciate its potential in ai development and its ability to advance the field of autonomous systems.
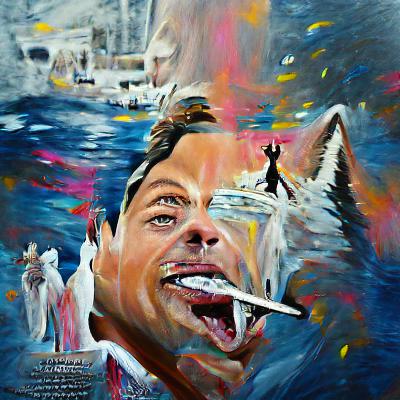
Credit: opensea.io
Analyzing The Key Components And Techniques
Understanding Imitation Learning – How Ai Agents Mimic Behavior
Imitation learning is a powerful technique in the field of artificial intelligence, enabling ai agents to mimic the behavior of human experts. By learning from demonstrations and imitating expert actions, these agents can quickly acquire complex skills and perform tasks with impressive efficiency.
In this section, we will analyze the key components and techniques involved in imitation learning.
Importance Of Data Collection And Annotation
- Collecting high-quality data: The first step in imitation learning is to gather a diverse and well-annotated dataset. This dataset consists of demonstrations performed by human experts, showcasing the desired behavior that the ai agent should emulate.
- Annotating the data: Once the data is collected, it needs to be accurately annotated. Annotation involves labeling the key elements and actions within each demonstration, enabling the ai agent to understand the context and extract meaningful information.
- Ensuring data diversity: It is crucial to include a wide range of demonstrations in the dataset to account for various scenarios and challenges. This diversity enhances the ability of ai agents to generalize and adapt their behavior in different situations.
Role Of Expert Demonstrations
- Learning from expert demonstrations: Expert demonstrations provide the foundation for imitation learning. By observing and analyzing the actions performed by human experts, ai agents can acquire valuable insights about the optimal strategies and decision-making processes.
- Extracting policies from demonstrations: During imitation learning, the ai agent learns to mimic expert policies by effectively modeling the observed behavior. By examining the demonstrations, the agent can identify the underlying patterns and formulate policies that guide its own actions.
- Balancing exploration and exploitation: While imitating expert demonstrations is beneficial, it is also crucial for the ai agent to explore alternative strategies. By striking a balance between exploration and exploitation, the agent can discover novel approaches and potentially improve upon the expert’s performance.
Evaluating Imitation Reward Functions
- Defining reward functions: In imitation learning, reward functions play a crucial role in shaping the ai agent’s behavior. These functions provide feedback and incentives to guide the agent towards desired outcomes. The reward functions need to be carefully designed to accurately reflect the expert’s behavior and the task’s objectives.
- Incorporating domain knowledge: To enhance the effectiveness of reward functions, incorporating domain knowledge can prove beneficial. By integrating prior knowledge of the task and its intricacies, the ai agent can navigate complex scenarios more intelligently and make informed decisions.
- Fine-tuning the reward functions: The evaluation and refinement of reward functions are continuous processes in imitation learning. Through iterative experimentation and analysis, the reward functions can be optimized, emphasizing the desired behavior and improving the agent’s performance.
Imitation learning is a valuable approach that allows ai agents to acquire complex skills by imitating expert behavior. By carefully collecting and annotating data, leveraging expert demonstrations, and fine-tuning reward functions, these agents can achieve impressive levels of performance. This understanding of the key components and techniques involved in imitation learning paves the way for the development of more intelligent and adaptive ai systems.
Leveraging Deep Learning And Reinforcement Learning
Imitation learning is a fascinating field within the realm of artificial intelligence. By mimicking or imitating human behavior, ai agents can learn to perform complex tasks and solve problems. This blog post section will explore how imitation learning leverages deep learning and reinforcement learning techniques to enable ai agents to imitate behavior effectively.
Transfer Learning In Imitation Learning:
- Transfer learning plays a crucial role in imitation learning as it allows ai agents to leverage knowledge learned from one task to another.
- By applying transfer learning, ai agents can benefit from pre-existing knowledge and adapt it to imitate new behaviors.
- Transfer learning helps in training ai agents faster by utilizing the knowledge learned from similar tasks.
Incorporating Reinforcement Learning In Imitation Learning:
- Reinforcement learning complements imitation learning by enabling ai agents to refine their learned behavior through trial and error.
- By incorporating reinforcement learning, ai agents can learn from their own experiences and adapt their behavior to achieve better performance.
- Reinforcement learning provides a mechanism for ai agents to actively explore and discover optimal strategies on their own.
Enhancing Mimicry With Generative Adversarial Networks:
- Generative adversarial networks (gans) have shown great promise in improving the mimicry capabilities of ai agents.
- Gans consist of a generator network and a discriminator network that work together to generate realistic and diverse imitations.
- The generator network generates imitated behavior, while the discriminator network evaluates the quality of the imitation, providing feedback for further improvement.
Leveraging deep learning and reinforcement learning techniques in imitation learning paves the way for ai agents to effectively mimic human behavior. Transfer learning helps in quickly adapting knowledge from similar tasks, while reinforcement learning enables agents to refine their behavior through self-exploration.
By incorporating generative adversarial networks, the mimicry abilities of ai agents are further enhanced, leading to more realistic and diverse imitations. With continuous advancements in these techniques, imitation learning holds great promise for various applications where imitating human behavior is crucial.
Frequently Asked Questions Of Understanding Imitation Learning – How Ai Agents Mimic Behavior
What Is Imitation Learning?
Imitation learning is a technique used by ai agents to mimic human behavior and learn from demonstrations.
How Do Ai Agents Mimic Behavior?
Ai agents mimic behavior by observing and imitating actions performed by humans or expert demonstrations.
Why Is Imitation Learning Important?
Imitation learning is important as it allows ai agents to learn complex behaviors from experts and improve their performance.
What Are The Applications Of Imitation Learning?
Imitation learning has various applications, including autonomous driving, robotics, and virtual assistants, enabling smarter and more human-like interactions.
How Does Imitation Learning Benefit Ai Development?
Imitation learning accelerates ai development by reducing the need for manual programming, allowing ai agents to learn from human expertise.
Conclusion
Imitation learning is an incredibly powerful technique in the world of ai. By allowing ai agents to mimic human behavior, we can achieve impressive results in various fields such as robotics, autonomous vehicles, and gaming. This approach leverages the vast amount of human knowledge and expertise, enabling machines to navigate and make decisions in real-world scenarios more effectively.
Imitation learning opens up exciting possibilities for ai agents to learn from human demonstrations, resulting in faster and more efficient training. With the ability to observe and imitate human behavior, machines can quickly adapt and make informed decisions in complex environments.
This technique also reduces the burden of hand-coding explicit instructions for every task, making it a valuable tool for developing intelligent systems. As ai continues to evolve, understanding and harnessing the power of imitation learning will be crucial in unlocking its full potential.
By bridging the gap between human and machine behavior, we can create ai agents that not only mimic but also understand and improve upon human performance. With further advancements, imitation learning is set to transform various industries, leading to safer, more efficient, and highly intelligent ai systems.